
Finding genetic variants responsible for human disease hiding in universe of benign variants
Klaus Lehnert and Russell Snell, Jessie Jacobsen and Brendan Swan, School of Biological Sciences
Introduction and research
Our programs aim to unravel the genetic basis of human diseases, using new approaches enabled by recent step-changes in genetic sequencing technologies (aka the “$1000 genome”). The human genome comprises 3 billion loci, and individuals typically differ from this ‘reference’ at millions of sites. These differences are the result of a complex interplay between ancient mutations, selection for survival fitness, mating between populations, events of near-extinction, and strong population expansion over the last ~150 years. A constant supply of new mutations creates new variants that are extremely rare. Some of these variants are directly responsible for disease, and others cause genetic diseases in unknown combinations.
We combine classical genetics approaches with genome sequencing to identify potentially disease-causing variants for experimental validation. One of our focus areas are neurological diseases, and we started a large project to understand the genetic nature of autism- spectrum disorders (www.mindsforminds.org.nz/), an often debilitating neurodevelopmental condition with increasing prevalence in all human populations. We expect that identification of genetic mutations will help us to better understand the disease process and identify new targets for therapeutic intervention. We take similar approaches to support mutation discovery in clinical research in collaboration with ADHB.
This is ’big data’ research – we typically process 100 billion ‘data points’ for each family, and the data analysis and storage requirements have pose significant challenges to traditionally data-poor biomedical analysis.
What was done on the Pan cluster and how it helped
Using the parallel processing options available on the Pan cluster we were able to derive optimal combinations for multiple inter- dependent parameters to align billions of short sequence reads to the human genome. These sequence read are strings of 100 nucleotide ‘characters’ (one of the four DNA ‘bases’ plus ‘not known’), including a confidence score for each base call, and contain a small number of differences to the reference string – the patient’s individual and population-specific variants. The non-uniform nature of the human genome reference further complicates the similarity search. The goal is to assign a unique position for each read in the genome, using mixed algorithms employing string matching to ‘seed’ the alignment and a combination of string matching and similarity scoring to extend the alignment through gaps and differences, taking into account the confidence score for each base call. Through parameter optimisation and multi-threading we successfully reduced run times from several hours to seven minutes per patient.
The second step of each patient’s genome analysis aims to derive ‘genotypes’ for each of the millions of loci that differ from the reference in each patient.
A genotype is a ‘best call’ for the two characters that can be observed at a single position (humans have two non-identical copies of each gene, one inherited from each parent). Genome sequencing creates 20-500 individual ‘observations’ for each of the 3 billion genome positions. The observations may be inconsistent, and/or may be different to the reference. We obtain a ‘consensus call’ for each position through a process that first proposes a de novo solution for the variant locus (i.e. not influenced by the reference), and then applies complex Bayesian framework to compute the most probable genotype at each locus.
This process requires approximately 2000 hours of computation for a single family. However, on the Pan cluster we can apply a classic scatter-gather approach: we split the genome into dozens of segments, compute genotypes and probabilities for all segments in parallel, and then combine the results from the individual computations to generate a list of all variants in each individual. Total compute time remains unchanged, but the process completes within a few hours!
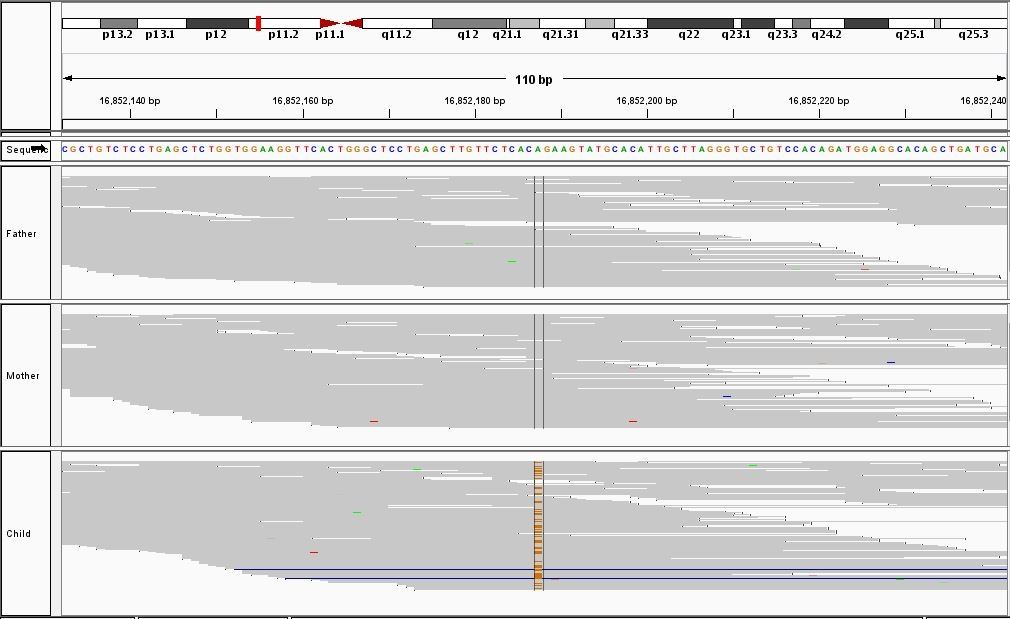
Figure 1: A new mutation – is it real, and does it cause disease? A visualisation of the alignment of 360 million 100-nucleotide reads for a parent-child trio against an unrelated genome reference. The window is zoomed on 110 of the 3 billion bases in the human genome (horizontal axis), the vertical axis shows features in the reference genome (top), the reference sequence (colour-coded), followed by three panels displaying several hundred reads (grey lines) obtained from the genomic DNA from father, mother, and child. Differences to the reference sequence are indicated in colour, positions identical to the reference are in grey (majority). Our analysis on Pan has clearly identified a new mutation in the child (orange squares, bottom panel), and it appears to affect only one of the child’s two gene copies.
We use standard bioinformatics software programs such as Burrows-Wheeler aligner, the Genome Analysis Toolkit (GATK), samtools, and others; these work very well but require multi- dimensional parameter optimisation. Being able to obtain results in hours instead of months allows us to analyse more patients than otherwise possible, and the time gained for variant interpretation increases the number of cases we can analyse. The NeSI approach has allowed us to perform this research in collaboration with colleagues in Christchurch and Dunedin. The Pan cluster is the perfect home for this collaboration, and the use of a shared computation platform leverages the development effort in the three research teams.
What’s next?
NeSI’s high-bandwidth connection and compute facilities will allow us to extend our collaboration across the Tasman, and we look forward to working with new collaborators in Australia. And of course there are disease-causing variants to be discovered!
See more case study projects

Our Voices: using innovative techniques to collect, analyse and amplify the lived experiences of young people in Aotearoa
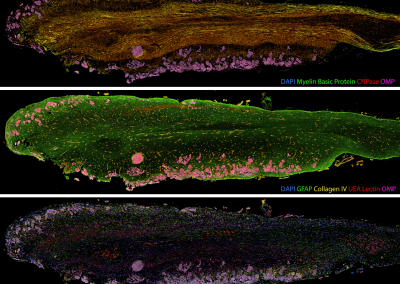
Painting the brain: multiplexed tissue labelling of human brain tissue to facilitate discoveries in neuroanatomy
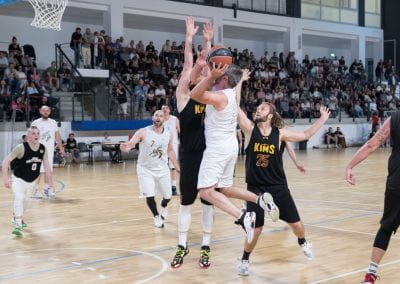
Detecting anomalous matches in professional sports: a novel approach using advanced anomaly detection techniques
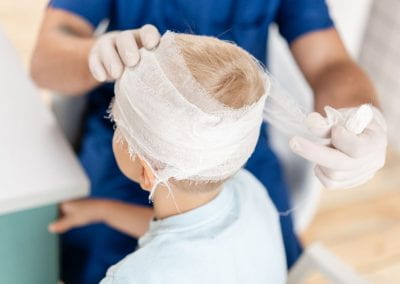
Benefits of linking routine medical records to the GUiNZ longitudinal birth cohort: Childhood injury predictors

Using a virtual machine-based machine learning algorithm to obtain comprehensive behavioural information in an in vivo Alzheimer’s disease model

Mapping livability: the “15-minute city” concept for car-dependent districts in Auckland, New Zealand

Travelling Heads – Measuring Reproducibility and Repeatability of Magnetic Resonance Imaging in Dementia
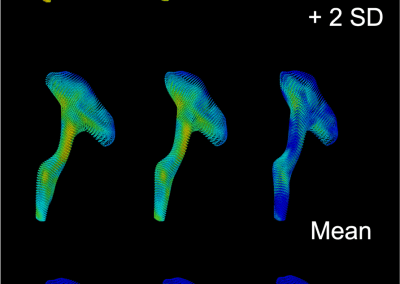
Novel Subject-Specific Method of Visualising Group Differences from Multiple DTI Metrics without Averaging

Re-assess urban spaces under COVID-19 impact: sensing Auckland social ‘hotspots’ with mobile location data

Aotearoa New Zealand’s changing coastline – Resilience to Nature’s Challenges (National Science Challenge)

Proteins under a computational microscope: designing in-silico strategies to understand and develop molecular functionalities in Life Sciences and Engineering
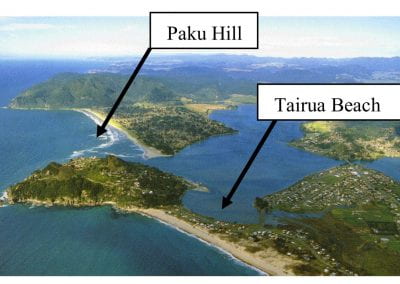
Coastal image classification and nalysis based on convolutional neural betworks and pattern recognition

Determinants of translation efficiency in the evolutionarily-divergent protist Trichomonas vaginalis
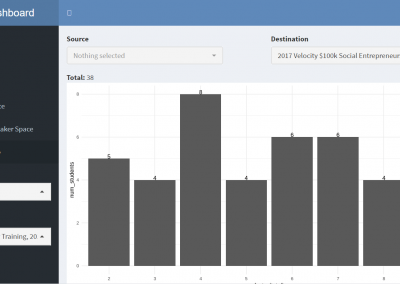
Measuring impact of entrepreneurship activities on students’ mindset, capabilities and entrepreneurial intentions
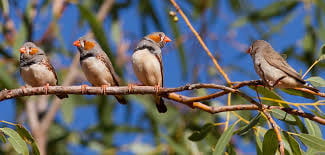
Using Zebra Finch data and deep learning classification to identify individual bird calls from audio recordings
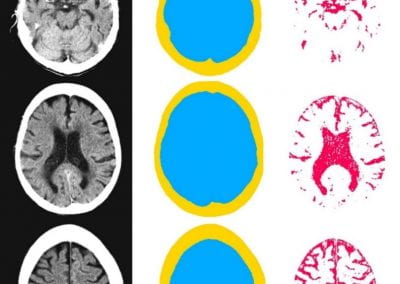
Automated measurement of intracranial cerebrospinal fluid volume and outcome after endovascular thrombectomy for ischemic stroke
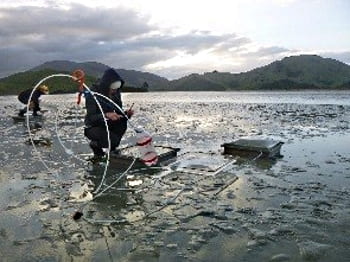
Using simple models to explore complex dynamics: A case study of macomona liliana (wedge-shell) and nutrient variations
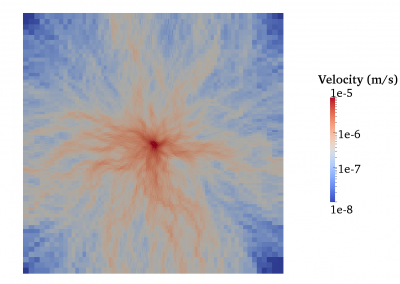
Fully coupled thermo-hydro-mechanical modelling of permeability enhancement by the finite element method
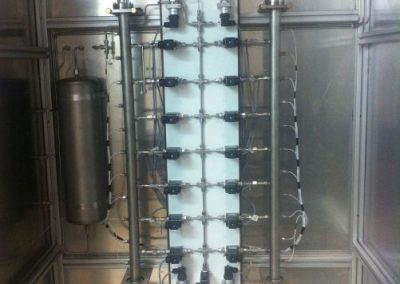
Modelling dual reflux pressure swing adsorption (DR-PSA) units for gas separation in natural gas processing
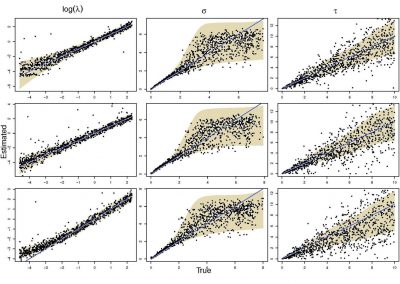
Molecular phylogenetics uses genetic data to reconstruct the evolutionary history of individuals, populations or species
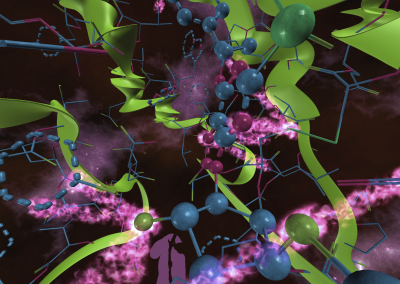
Wandering around the molecular landscape: embracing virtual reality as a research showcasing outreach and teaching tool
