
Development of Machine Learning methodology for genomic research
Irene Leathwick, PhD Candidate, Professor Mark Gahegan, Supervisor, School of Computer Science; Associate Professor Klaus Lehnert, Co-Supervisor, School of Biological Sciences,

Introduction
Recent development in computational power and generation of large quantity of data have resulted in an explosion of interest and application for Machine Learning (ML) and Deep Learning (DL) techniques. Many fields are experiencing the impact of this, primarily those in which large data are becoming available. ML is now able to uncover complex patterns which human would find them challenging and difficult to conceive. It has achieved astounding results in many areas, such as medical diagnostics, language translation, and driverless cars, amongst other areas.
Study of genomic prediction
This research project focuses on developing and modifying artificial intelligence (AI) tools, namely ML and DL tools, for genetic data. Out of the 3 billion bases that compose the whole genome of a cow or human, 20-25 million are different between individuals. Of these. Some genetic differences are beneficial and result in desirable traits; others contribute to less desirable outcomes such as disease. Artificial intelligence can help us interpret raw genetic data and improve our understanding of how the genetic differences influence outcomes, whether that be traits like eye colour, or disease. The goal is to develop a methodology to be more accurately mapping their genetic differences to their traits.
Up to recently, statistical methods are used to predict which genetic differences affect which traits. One of the limitations is that these methods are only feasible with a very small portion of the total genetic data (about 0.0000001%). Another limitation is that they are unsuitable for handling the relationship between complex genetic to trait (such as multiple genes affecting one trait).
Machine Learning and Deep Learning
The application of artificial intelligence methods to genetic data is very novel and only beginning to be explored, because only recently has the cost of obtaining genetic data allowed for sufficient data to be produced. The ML/DL methods are able to handle such large quantities of data, seem apt for extracting complex associations between genetic variations and traits.
The applicability of this research is wide, being useful anywhere where genetic data is used to predict traits. It could help with diagnosis of diseases, especially those that are rare and are hard to predict by traditional methods. It can benefit precision medicine, the movement to tailor treatments based on an individual’s genetic makeup. In animal breeding, it can help make predictions for characteristics in animals that have economic value, and increase their performance with each generation. Publications from this research may also benefit conservation efforts, by predicting human effects on biodiversity, predicting the spread of invasive species and outcomes of countermeasures, and answering other questions in ecology.
Work up-to-date and future plans
This work is part of a PhD and is currently in its first year. Some of the work I’ve implemented and explored are outlined below:
- Using simple convolutional neural networks (CNNs) for genomic variant calling. Variant calling is a routine first step for many analyses. There is a set of sequences for each species that act as the “reference” for genomic analysis work. Since the genetic sequence is mostly identical from person to person, an economic way of finding useful information about an individual’s genetic information is to identify the small differences from the reference genome. CNNs are excellent at image recognition, and have been used to try and improve upon the accuracy of variant detection treating DNA like a long, four-pixel wide image.
- Using CNNs to find splice site boundaries. Every gene is made out of exons (which are expressed) and introns (which are discarded), but there may be a different combination of exons that become expressed in any one individual. Some of these differences lie at the heart of disease. It has therefore been an important goal to find mutations that result in different splice site boundaries, and to prioritize them for further research. Based on work published this year, I have been exploring hyperparameter optimisation and different input structures to this CNN.
- Using Generative Adversarial Networks (GANs) to detect splice site boundaries, and produce novel DNA sequences with desired properties. Here, we train two networks in conjunction with one another: the discriminator learns to detect splice sites, and to flag sequences as fake, if it thinks any were made by the generator, which is another network that learns to fool the discriminator. Throughout this process, the discriminator gets better and better at learning what a real splice site looks like, while the generator learns how to make realistic looking DNA sequences that won’t be detected as fake by the discriminator.
- While individual predictors have their strengths and weaknesses, there has been little research to do with combining predictors together so that they can compensate for each other’s weaknesses. The next step in this research will be to look at combining predictors to tackle the same learning task, which may improve predictive power as well as give insight into what each learner struggles with.
Acknowledgement
The author wishes to thank Sina Masoud-Ansari, Senior Solutions Special from the Centre for eResearch for his technical support in accessing to a Tesla V100. The author uses NeSI HPC platform for hyperparameter tuning experiments. Sina has also helped with Tensorflow/keras packages which are working on both AWS and NeSI.
See more case study projects

Our Voices: using innovative techniques to collect, analyse and amplify the lived experiences of young people in Aotearoa
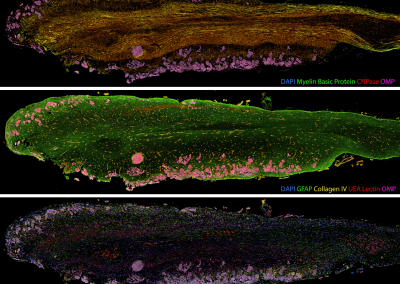
Painting the brain: multiplexed tissue labelling of human brain tissue to facilitate discoveries in neuroanatomy
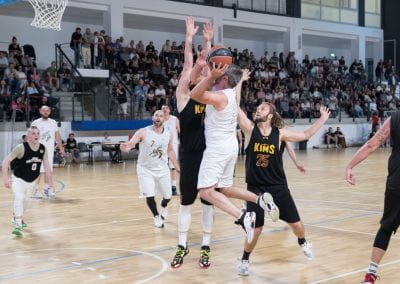
Detecting anomalous matches in professional sports: a novel approach using advanced anomaly detection techniques
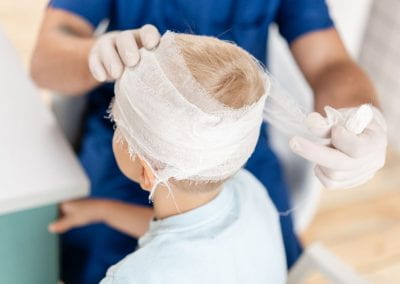
Benefits of linking routine medical records to the GUiNZ longitudinal birth cohort: Childhood injury predictors

Using a virtual machine-based machine learning algorithm to obtain comprehensive behavioural information in an in vivo Alzheimer’s disease model

Mapping livability: the “15-minute city” concept for car-dependent districts in Auckland, New Zealand

Travelling Heads – Measuring Reproducibility and Repeatability of Magnetic Resonance Imaging in Dementia
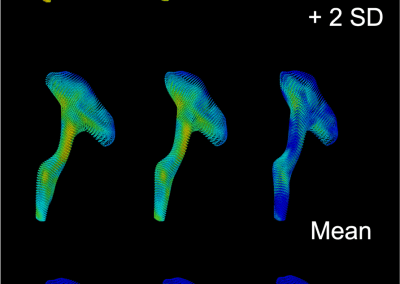
Novel Subject-Specific Method of Visualising Group Differences from Multiple DTI Metrics without Averaging

Re-assess urban spaces under COVID-19 impact: sensing Auckland social ‘hotspots’ with mobile location data

Aotearoa New Zealand’s changing coastline – Resilience to Nature’s Challenges (National Science Challenge)

Proteins under a computational microscope: designing in-silico strategies to understand and develop molecular functionalities in Life Sciences and Engineering
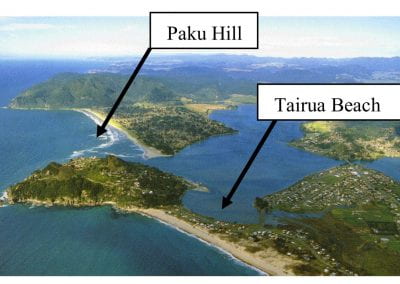
Coastal image classification and nalysis based on convolutional neural betworks and pattern recognition

Determinants of translation efficiency in the evolutionarily-divergent protist Trichomonas vaginalis
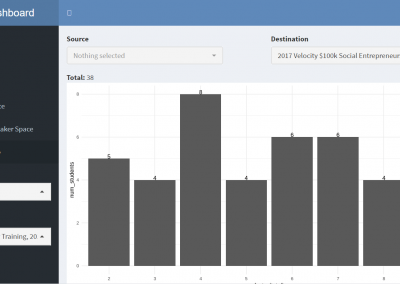
Measuring impact of entrepreneurship activities on students’ mindset, capabilities and entrepreneurial intentions
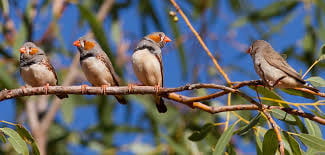
Using Zebra Finch data and deep learning classification to identify individual bird calls from audio recordings
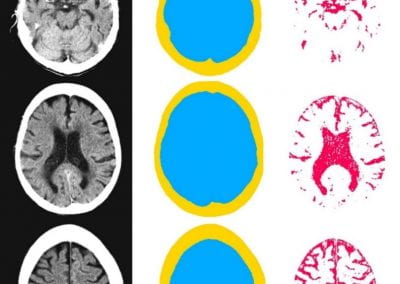
Automated measurement of intracranial cerebrospinal fluid volume and outcome after endovascular thrombectomy for ischemic stroke
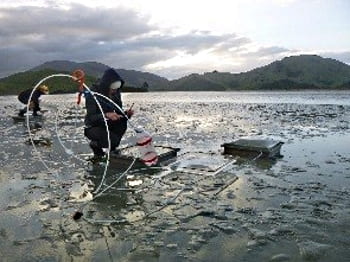
Using simple models to explore complex dynamics: A case study of macomona liliana (wedge-shell) and nutrient variations
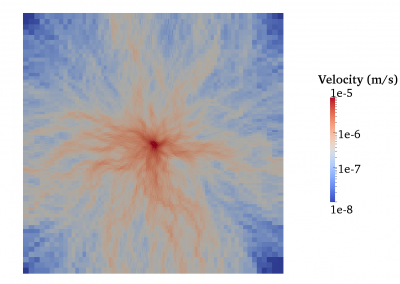
Fully coupled thermo-hydro-mechanical modelling of permeability enhancement by the finite element method
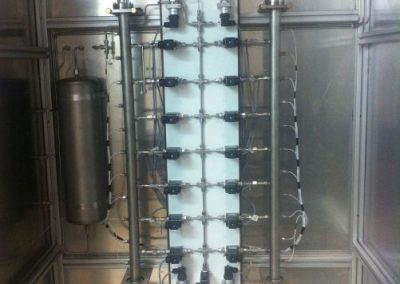
Modelling dual reflux pressure swing adsorption (DR-PSA) units for gas separation in natural gas processing
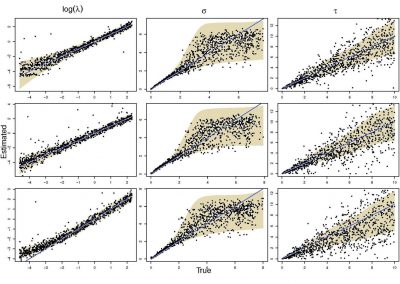
Molecular phylogenetics uses genetic data to reconstruct the evolutionary history of individuals, populations or species
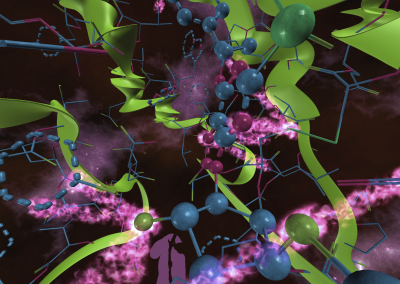
Wandering around the molecular landscape: embracing virtual reality as a research showcasing outreach and teaching tool
