
Estimating migration rates in the budding yeast Saccharomyces cerevisiae
Sarah Knight, School of Biological Sciences
Background
Eukaryotic microbes are key ecosystem drivers, but we have little theory and few data elucidating the processes influencing their observed population patterns. Because of their large population sizes, and ease of transfer, one might expect microbial populations to be well mixed, but there is increasing evidence showing that many are not homogeneous but structured. Understanding the processes that drive population structure and connectivity has implications for understanding the evolutionary trajectories of these organisms.
The budding yeast Saccharomyces cerevisiae is of significant commercial importance due to its role in the production of bread, wine, beer and other alcoholic beverages but is also widely used by the scientific community as a model research organism. While we have a vast knowledge of its cell biology, genetics and increasingly its ecology and evolution, studies of its population patterns are often confounded by geography.
Since microbes are inherently difficult to observe directly, we rely on genetic methods to infer their movements. As part of a larger investigation into the population dynamics of S. cerevisiae in New Zealand, we sampled S. cerevisiae from vineyards and surrounding native bush in major Sauvignon Blanc growing regions and genotyped 850 individual isolates at eight microsatellite loci.
After removing identical genotypes from the same samples due to the clonal nature of growth of S. cerevisiae during the culturing process, the final dataset comprised 369 microsatellite profiles. This dataset was used to infer patterns of population structure and connectivity between these regions and between managed and unmanaged ecosystems within each region (*Knight and Goddard, In Press).

Figure 1: Adapted from *Knight and Goddard (In press). Migration rates between regions as calculated by MIGRATE.
*This research has recently been accepted for publication by The ISME Journal.
Estimating migrations rates using the Pan cluster
Analyses of genetic data to infer migration rates are typically very computationally expensive. We used a Bayesian coalescent approach implemented in MIGRATE (Beerli, 2006; Beerli, 2009) that ran ten replicate MCMC chains of one million steps in length across all eight loci. With the help of staff at NeSI, optimal settings were determined and we managed to run each chain for each locus in parallel, essentially reducing the time it took to run the analysis by 80 times! Even then the analysis still took weeks to run and without the resources provided by NeSI this analysis would not have been possible.
What’s next?
Our results suggest that migration of S. cerevisiae in New Zealand mimics the movements of fruit by the wine industry, with larger migration rates into the larger wine producing regions. While this work is now largely completed, it would be interesting to examine what differences exist in the population dynamics of microbial eukaryotes that do not have such a close association with human activity. Can we still detect human influenced dispersal? Or are these populations more highly structured?
- Beerli, P. (2006). Comparison of Bayesian and maximum-likelihood inference of population genetic parameters. Bioinformatics 22(3): 341-345.
- Beerli, P. (2009). How to use MIGRATE or why are Markov chain Monte Carlo programs difficult to use? Population Genetics for Animal Conservation. G. Bertorelle, M. W. Bruford, H. C. Hauffe, A. Rizzoli and C. Vernesi. New York, USA, Cambridge University Press: 42-79.
See more case study projects

Our Voices: using innovative techniques to collect, analyse and amplify the lived experiences of young people in Aotearoa
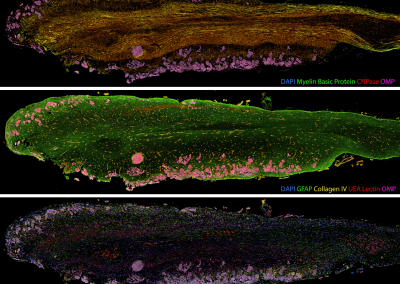
Painting the brain: multiplexed tissue labelling of human brain tissue to facilitate discoveries in neuroanatomy
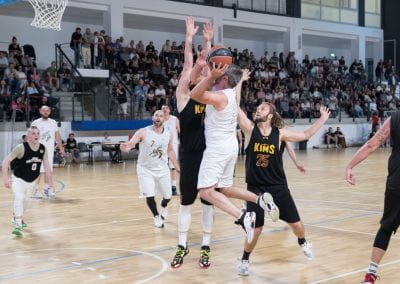
Detecting anomalous matches in professional sports: a novel approach using advanced anomaly detection techniques
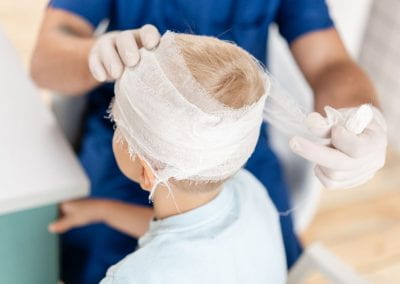
Benefits of linking routine medical records to the GUiNZ longitudinal birth cohort: Childhood injury predictors

Using a virtual machine-based machine learning algorithm to obtain comprehensive behavioural information in an in vivo Alzheimer’s disease model

Mapping livability: the “15-minute city” concept for car-dependent districts in Auckland, New Zealand

Travelling Heads – Measuring Reproducibility and Repeatability of Magnetic Resonance Imaging in Dementia
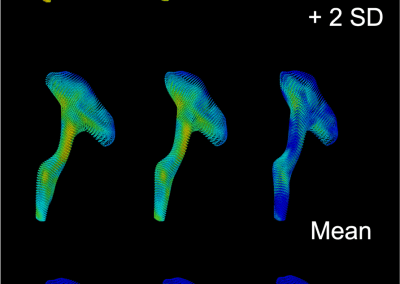
Novel Subject-Specific Method of Visualising Group Differences from Multiple DTI Metrics without Averaging

Re-assess urban spaces under COVID-19 impact: sensing Auckland social ‘hotspots’ with mobile location data

Aotearoa New Zealand’s changing coastline – Resilience to Nature’s Challenges (National Science Challenge)

Proteins under a computational microscope: designing in-silico strategies to understand and develop molecular functionalities in Life Sciences and Engineering
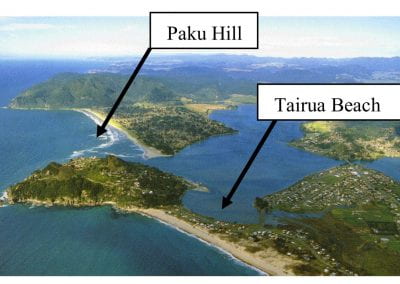
Coastal image classification and nalysis based on convolutional neural betworks and pattern recognition

Determinants of translation efficiency in the evolutionarily-divergent protist Trichomonas vaginalis
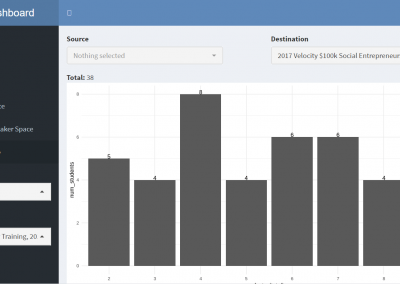
Measuring impact of entrepreneurship activities on students’ mindset, capabilities and entrepreneurial intentions
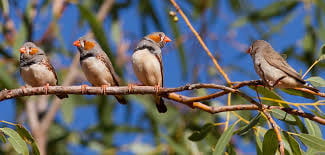
Using Zebra Finch data and deep learning classification to identify individual bird calls from audio recordings
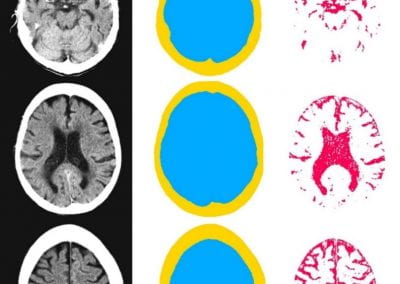
Automated measurement of intracranial cerebrospinal fluid volume and outcome after endovascular thrombectomy for ischemic stroke
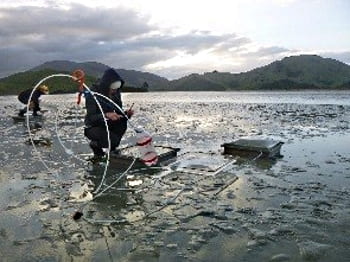
Using simple models to explore complex dynamics: A case study of macomona liliana (wedge-shell) and nutrient variations
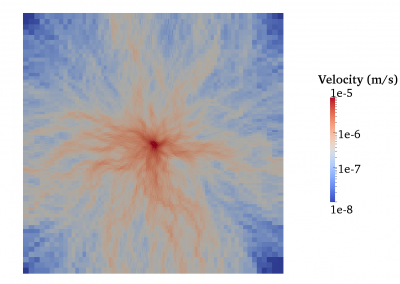
Fully coupled thermo-hydro-mechanical modelling of permeability enhancement by the finite element method
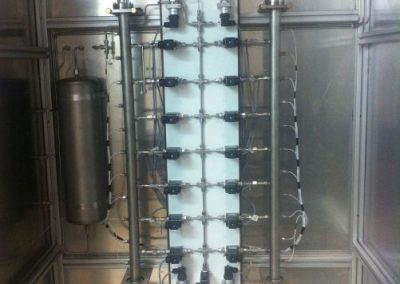
Modelling dual reflux pressure swing adsorption (DR-PSA) units for gas separation in natural gas processing
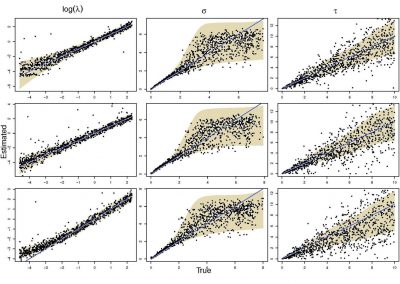
Molecular phylogenetics uses genetic data to reconstruct the evolutionary history of individuals, populations or species
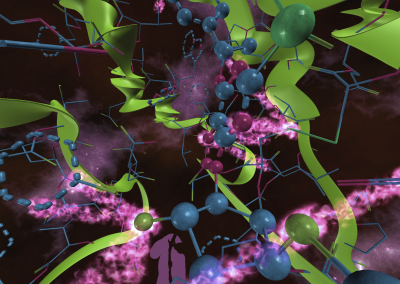
Wandering around the molecular landscape: embracing virtual reality as a research showcasing outreach and teaching tool
