
Improving In Vitro Fertilisation (IVF) with Machine and Deep Learning
Dr Nicholas Knowlton, Senior Research Fellow, Molecular Medicine and Pathology, Faculty of Medical and Health Sciences;
Dr Nidhi Gowdra, eResearch Solutions Specialist, Centre for eResearch
By exploring how to improve the success rate of In Vitro Fertilisation (IVF) implantations, we hope the knowledge will be embedded in a model and made it widerly available locally and overseas where the investment will generate export value for New Zealand and benefit the needed parents by reducing IVF waiting time and increasing the rate of live births.
The current process of selecting embryos for implantation in IVF is based on little knowledge of the relationship between the parameters for embryo selection and the actual success rates post-implantation. Embryos are selected based on the features in a single image taken at a single time. Some researchers and clinicians are starting to apply artificial intelligence (AI) to a selection of embryos, thereby considering multiple factors at once that indicate potential successful implantation and live birth more likely. Unfortunately, most of these schemes try to copy embryologists’ current and limited approach. We have evaluated the existing schemes and realised that we can do much better; particularly given that none of them appear to improve success rates.
Our team of an embryo quality specialist, a machine learning/AI expert, and a clinical embryologist, a key opinion leader in embryology, will use exclusive access to billions of embryo images alongside their clinical information to develop an AI-based approach to embryo selection. We will use information regarding a wide range of aspects of the embryo at different stages in its development, together with information regarding the parents. This knowledge will be embedded in a model, which will be made available widely in New Zealand and overseas by a new company developed for the purpose. This enterprise will create new export returns for NZ through selling access to the model while generating significant social benefits in New Zealand by reducing IVF waiting times and increasing the number of live births from IVF.
The Centre for eResearch is a key partner in this project through their Machine and Deep Learning (MaD) Service. They provide support with data storage, retrieval, organization, integration with third-party tools and direct coding support. By leveraging their expertise with my research group, we can work more efficiently by tapping into a much larger experience base. While the project is still ongoing, we will discuss our progress on automated embryo morphokinetic identification in this project update.
Problem
Lay method description
Technical method description
Results
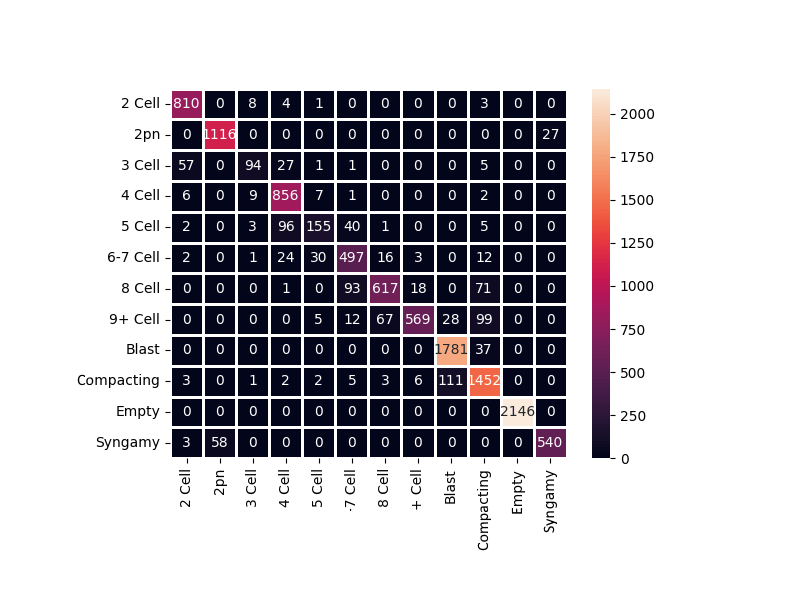
See more case study projects

Our Voices: using innovative techniques to collect, analyse and amplify the lived experiences of young people in Aotearoa
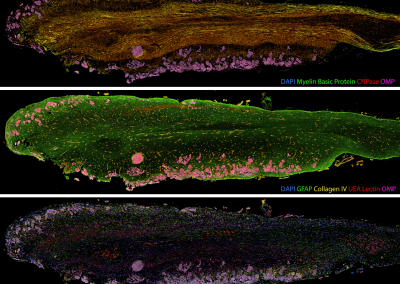
Painting the brain: multiplexed tissue labelling of human brain tissue to facilitate discoveries in neuroanatomy
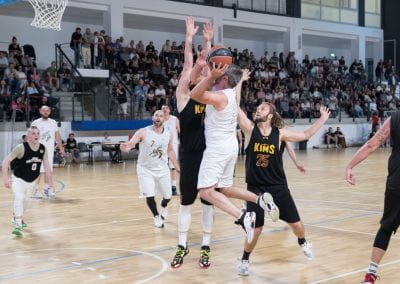
Detecting anomalous matches in professional sports: a novel approach using advanced anomaly detection techniques
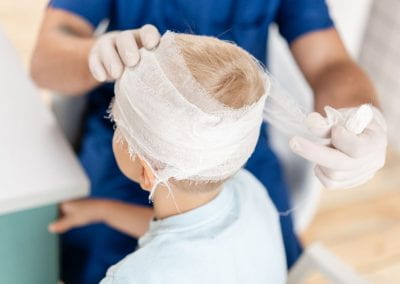
Benefits of linking routine medical records to the GUiNZ longitudinal birth cohort: Childhood injury predictors

Using a virtual machine-based machine learning algorithm to obtain comprehensive behavioural information in an in vivo Alzheimer’s disease model

Mapping livability: the “15-minute city” concept for car-dependent districts in Auckland, New Zealand

Travelling Heads – Measuring Reproducibility and Repeatability of Magnetic Resonance Imaging in Dementia
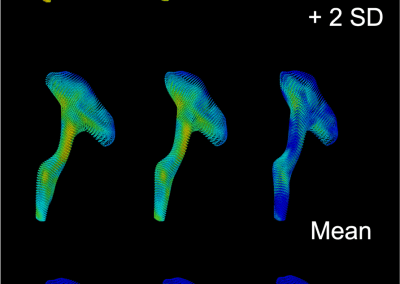
Novel Subject-Specific Method of Visualising Group Differences from Multiple DTI Metrics without Averaging

Re-assess urban spaces under COVID-19 impact: sensing Auckland social ‘hotspots’ with mobile location data

Aotearoa New Zealand’s changing coastline – Resilience to Nature’s Challenges (National Science Challenge)

Proteins under a computational microscope: designing in-silico strategies to understand and develop molecular functionalities in Life Sciences and Engineering
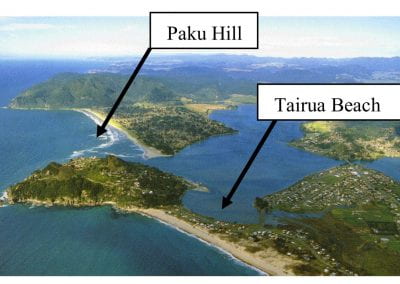
Coastal image classification and nalysis based on convolutional neural betworks and pattern recognition

Determinants of translation efficiency in the evolutionarily-divergent protist Trichomonas vaginalis
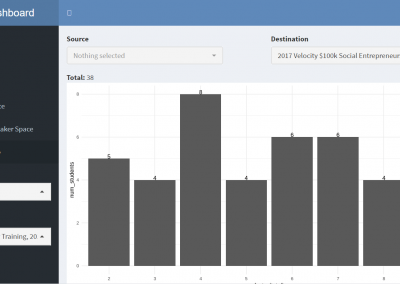
Measuring impact of entrepreneurship activities on students’ mindset, capabilities and entrepreneurial intentions
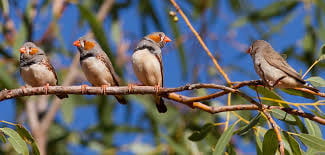
Using Zebra Finch data and deep learning classification to identify individual bird calls from audio recordings
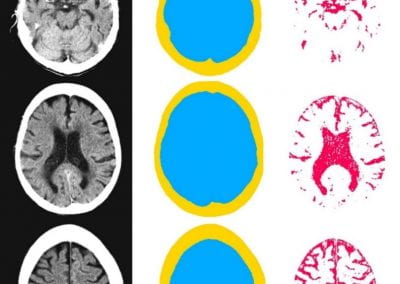
Automated measurement of intracranial cerebrospinal fluid volume and outcome after endovascular thrombectomy for ischemic stroke
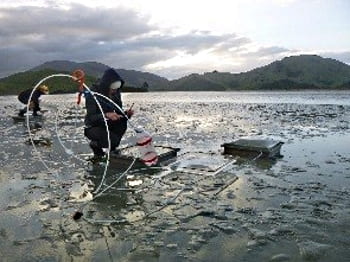
Using simple models to explore complex dynamics: A case study of macomona liliana (wedge-shell) and nutrient variations
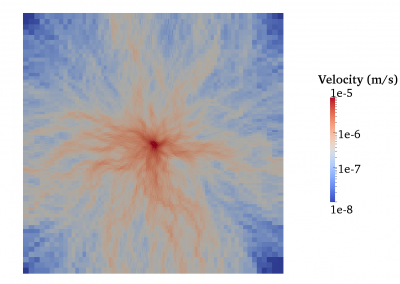
Fully coupled thermo-hydro-mechanical modelling of permeability enhancement by the finite element method
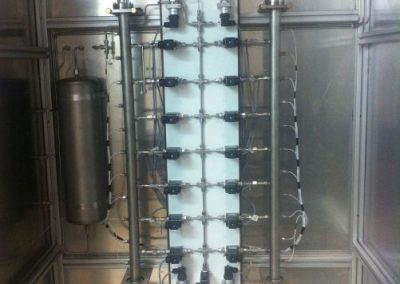
Modelling dual reflux pressure swing adsorption (DR-PSA) units for gas separation in natural gas processing
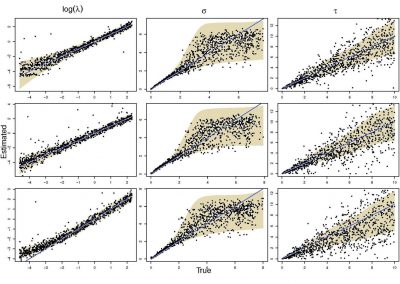
Molecular phylogenetics uses genetic data to reconstruct the evolutionary history of individuals, populations or species
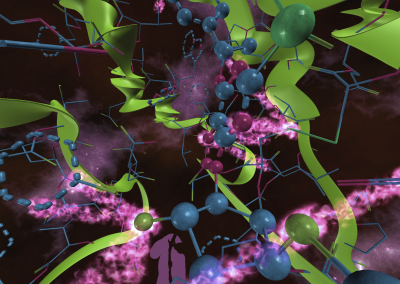
Wandering around the molecular landscape: embracing virtual reality as a research showcasing outreach and teaching tool
