
Interpretation of Non-coding Mutations Driving Melanoma Risk and Its Comorbidities
Michael Pudjihartono, PhD Candidate, Dr. William Schierding, Senior Research Fellow, Prof Justin O’Sullivan, Liggins Institute
Background
Melanoma is the deadliest form of skin cancer with increasing worldwide incidence. Understanding the underlying mechanisms driving melanoma is crucial for better treatment and prevention. Over the past two decades, the field of genomics has entered a new golden age due to advancements in genetic sequencing and genotyping technologies, which have made genome-wide assessment of genetic mutations that associate with disease risk (i.e. Genome-wide Association Studies; GWAS) possible. However, the interpretation of GWAS remains a challenge because most Single Nucleotide Polymorphisms (SNPs) found to be associated with diseases, including melanoma, lie in the non-coding regions of the genome. These are regions of the genome which lie outside of genes and thus do not code for any proteins. Rather than affecting protein structure, it is hypothesized that modifying the expression level of distal target genes is one mode of possible mechanism by which these non-coding SNPs affect disease risk. In this study, we integrated publicly available data from melanoma GWAS with tissue-specific markers of the 3D DNA structure (Hi-C) and gene expression data (eQTLs) to identify the genes dysregulated by non-coding risk SNPs in three melanoma-relevant tissues: melanocytes, sun-exposed skin, and not sun-exposed skin. This approach essentially finds the connection from non-coding SNPs to genes, which help to paint a clearer picture on how non-coding SNPs can potentially contribute to the development of melanoma and its comorbidities.
Methods
GWAS data for melanoma was downloaded from the GWAS Catalog1. Data on 3D genome organization (Hi-C) was downloaded from ENCODE2. Expression quantitative trait loci (eQTL) data from melanocyte, sun-exposed skin and not sun-exposed skin was downloaded from GTEx3 and a previous study4. These data were then loaded into the CoDeS3D pipeline5. The CoDeS3D pipeline takes the GWAS SNPs as input and identify regulatory associations between each SNP and specific target genes that are supported by both physical interaction data (Hi-C) and expression data (eQTL) [Figure 1a]. These identified the final set of melanoma target genes. To identify other comorbid traits associated with melanoma, the melanoma target genes were used as input to identify every other SNPs in the genome that associate with the same set of target genes as melanoma [Figure 1b]. Hypergeometric tests (FDR≤0.05) and bootstrapping (n = 350) were then performed to identify enrichment of the SNPs within GWAS Catalog. This process was repeated separately using expression data from melanocyte, sun-exposed skin and not sun-exposed skin.

Results
Across the three melanoma relevant tissues, a total of 151 genes were identified as being regulated by melanoma GWAS risk SNPs [Figure 2a]. Functional profiling of the 151 target genes showed an enrichment of genes important for various biological processes including developmental processes, apoptosis, and pigmentation. These are consistent with the role of normal cell development, regulation of cell death, and UV damage in the risk of developing melanoma. The comorbidity landscape of melanoma includes a total of 64 significant traits across the three tissues [Figure 2b]. These include 1) Traits with obvious relevance to melanoma (e.g traits relating to cancer, nevus count, pigmentation and telomere length). 2) Traits that are supported by clinical and/or epidemiological observations (e.g increased intraocular pressure in patients with iris melanoma6, increased melanoma risk for patients with actinic keratosis7). And 3) traits that have not been (or weakly) associated with melanoma (e.g. uterine fibroids and spontaneous coronary artery dissection).
Notably, the comorbidity landscape of melanoma is facilitated by target genes that are tissue specific, with 98 genes (65% of target genes) specific to only one of the three tissue types [Figure 2a]. Nonetheless, the comorbid traits found in all three tissues largely agree with each other (e.g traits relating to cancer, pigmentation traits, telomere length, and nevus) [Figure 2b], suggesting a higher-level convergence at the phenotype and pathway level.
This study provides novel insights into the biological implications of non-coding SNPs associated with melanoma risk and provide a starting point for further experimental validation of functional variants and disease-related genes.

Figure 2. (a) Overlap of the target genes of melanoma risk SNPs found in each of the three tissues. (b) Melanoma comorbidity landscape found in each of the three tissues.
References
- Buniello A, Macarthur JAL, Cerezo M, et al. The NHGRI-EBI GWAS Catalog of published genome-wide association studies, targeted arrays and summary statistics 2019. Nucleic Acids Res. 2019;47(D1):D1005-D1012. doi:10.1093/nar/gky1120
- Dunham I, Kundaje A, Aldred SF, et al. An integrated encyclopedia of DNA elements in the human genome. Nature 2012 489:7414. 2012;489(7414):57-74. doi:10.1038/nature11247
- Aguet F, Barbeira AN, Bonazzola R, et al. The GTEx Consortium atlas of genetic regulatory effects across human tissues. Science (1979). 2020;369(6509):1318-1330. doi:10.1126/SCIENCE.AAZ1776
- Zhang T, Choi J, Kovacs MA, et al. Cell-type-specific eQTL of primary melanocytes facilitates identification of melanoma susceptibility genes. Genome Res. 2018;28(11):1621-1635. doi:10.1101/gr.233304.117
- Fadason T, Schierding W, Lumley T, O’Sullivan JM. Chromatin interactions and expression quantitative trait loci reveal genetic drivers of multimorbidities. Nat Commun. Published online 2018. doi:10.1038/s41467-018-07692-y
- Shields CL, Materin MA, Shields JA, Gershenbaum E, Singh AD, Smith A. Factors associated with elevated intraocular pressure in eyes with iris melanoma. Br J Ophthalmol. 2001;85(6):666-669. doi:10.1136/BJO.85.6.666
- Guorgis G, Anderson CD, Lyth J, Falk M. Actinic Keratosis Diagnosis and Increased Risk of Developing Skin Cancer: A 10-year Cohort Study of 17,651 Patients in Sweden. Acta Derm Venereol. 2020;100(8):1-6. doi:10.2340/00015555-3486
See more case study projects

Our Voices: using innovative techniques to collect, analyse and amplify the lived experiences of young people in Aotearoa
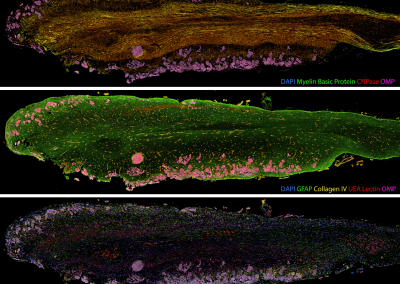
Painting the brain: multiplexed tissue labelling of human brain tissue to facilitate discoveries in neuroanatomy
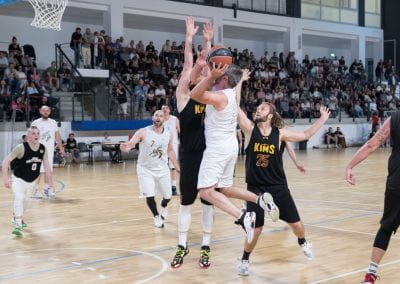
Detecting anomalous matches in professional sports: a novel approach using advanced anomaly detection techniques
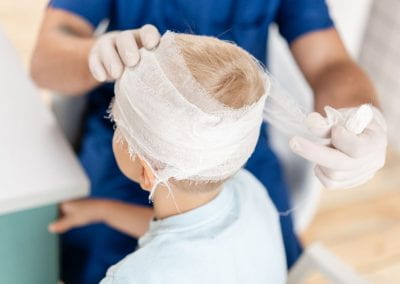
Benefits of linking routine medical records to the GUiNZ longitudinal birth cohort: Childhood injury predictors

Using a virtual machine-based machine learning algorithm to obtain comprehensive behavioural information in an in vivo Alzheimer’s disease model

Mapping livability: the “15-minute city” concept for car-dependent districts in Auckland, New Zealand

Travelling Heads – Measuring Reproducibility and Repeatability of Magnetic Resonance Imaging in Dementia
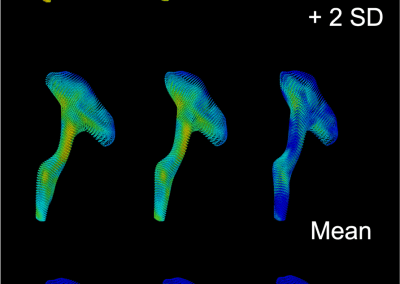
Novel Subject-Specific Method of Visualising Group Differences from Multiple DTI Metrics without Averaging

Re-assess urban spaces under COVID-19 impact: sensing Auckland social ‘hotspots’ with mobile location data

Aotearoa New Zealand’s changing coastline – Resilience to Nature’s Challenges (National Science Challenge)

Proteins under a computational microscope: designing in-silico strategies to understand and develop molecular functionalities in Life Sciences and Engineering
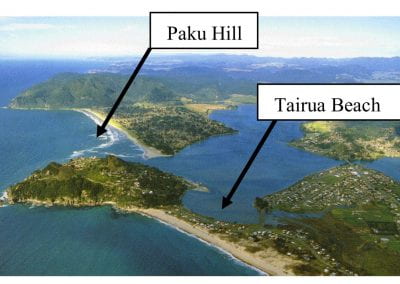
Coastal image classification and nalysis based on convolutional neural betworks and pattern recognition

Determinants of translation efficiency in the evolutionarily-divergent protist Trichomonas vaginalis
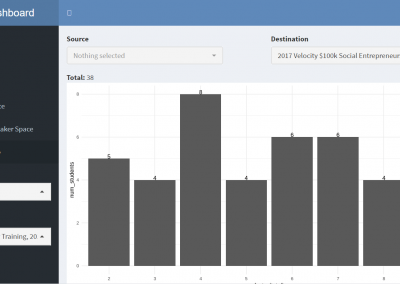
Measuring impact of entrepreneurship activities on students’ mindset, capabilities and entrepreneurial intentions
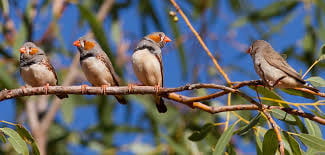
Using Zebra Finch data and deep learning classification to identify individual bird calls from audio recordings
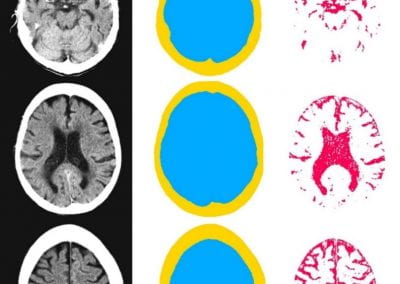
Automated measurement of intracranial cerebrospinal fluid volume and outcome after endovascular thrombectomy for ischemic stroke
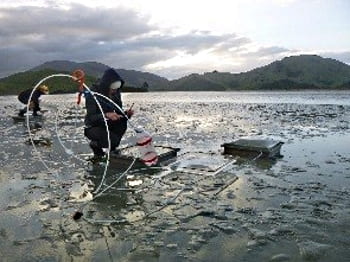
Using simple models to explore complex dynamics: A case study of macomona liliana (wedge-shell) and nutrient variations
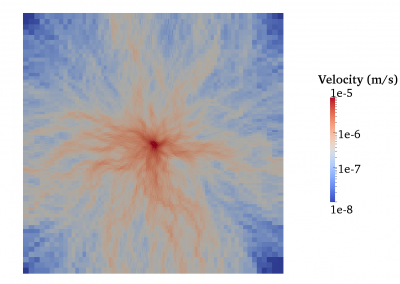
Fully coupled thermo-hydro-mechanical modelling of permeability enhancement by the finite element method
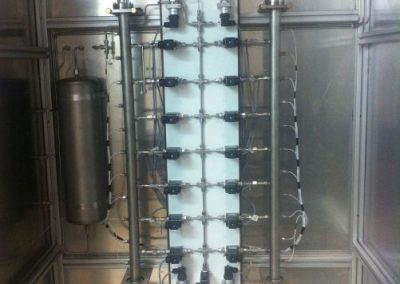
Modelling dual reflux pressure swing adsorption (DR-PSA) units for gas separation in natural gas processing
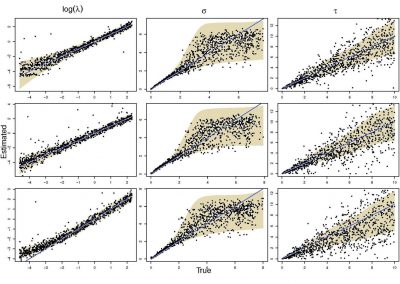
Molecular phylogenetics uses genetic data to reconstruct the evolutionary history of individuals, populations or species
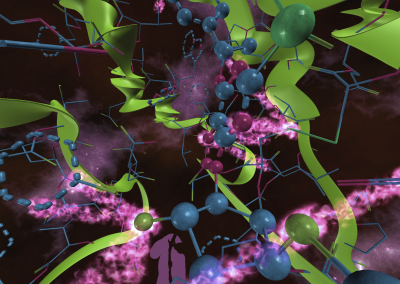
Wandering around the molecular landscape: embracing virtual reality as a research showcasing outreach and teaching tool
