
Proteins under a computational microscope: designing in-silico strategies to understand and develop molecular functionalities in Life Sciences and Engineering
Dr Davide Mercadante, Senior Lecturer, School of Chemical Sciences
One word to spell the future out loud
Over the last century, Biology has seen a steady increase in the number of macromolecular structures being resolved by means of either X-ray crystallography, NMR or, more recently, cryo-electron microscopy (cryo-EM). However, even since the early days it appeared clear that structure alone wasn’t the endpoint for investigating molecular behaviour. Antibody research in the 1930’s showed there were limitations to fully explaining molecular function by solely looking at structure as in order to bind a large diversity of molecules, the molecular structure of antibodies needs to contain a certain degree of plasticity. This suggested that looking at an ensemble of molecular conformations interconverting over time, rather than a single structure, was probably the best way to understand how molecules function. In other words, a key factor regulating molecular function is molecular dynamics, which experimental techniques have historically struggled to define at high-resolution. This has on the other hand been successfully tackled by computational approaches that have evolved extremely fast through developments in both algorithms and hardware.
The rapid evolution of molecular hardware has probably been the major developmental factor of the field, with scientists continuously eager to simulate larger molecular complexes, for longer time.
If you were to ask a computational biophysicist like me, to describe the future in a single word, you would probably receive the answer in the acronym GPU. Graphical processing units (GPUs), thanks to their high core density, have revolutionised the computation of molecular dynamics. By looking back just a few years we
Molecular dynamics spans timescales of several orders of magnitudes: from nanoseconds (a billionth of a second) to seconds and beyond. Before 2007, when nVidia introduced the first GPU cards, not only to accelerate graphical applications but also scientific simulations, few hundreds of nanoseconds would take several weeks to simulate. Today, in just a few days we can easily break the “wall of microseconds (μs)”, which is where many biological processes occur. Now we can more easily study molecular processes, design drugs to block or enhance cellular targets or even design new molecules that would tackle challenging problems from food safety to biomedics.
My group, which is a recent addition to the research landscape of New Zealand, has been built to fit the purpose of understanding and designing molecular function starting from the simulation of molecular dynamics.
By taking advantage of the computational infrastructure currently available at the University of Auckland via Nectar, we were able to start and progress several projects targeting a variety of purposes: from the improvement of food safety and the reduction of food allergenicity, to the design of sweet proteins to substitute artificial sweeteners. Below, I provide a brief overview of some of the projects currently undertaken by means of molecular simulations strictly interfaced with experiments, all of which have made great use of the Nectar cloud.
Molecular simulations in the cloud – from protein understanding to design
Re-design proteins to make them resist high temperatures
Proteins are the workhorses of cells and perform a myriad of different functions: from providing structural integrity to the cell to constantly transforming metabolites in a highly efficient manner (enzymes). They have evolved over millions of years to fulfil their function, which over time has become optimal for their individual micro-environment. Nevertheless, such a micro-environment does not often match the micro-environment in which industry could make good use of a protein function. A multitude of industrial processes are indeed carried out at temperatures well in excess of the thermal stability of proteins, leading to their inactivation.
We are therefore developing and applying a series of algorithms that look at the sequences of a multitude of proteins to redesign and target their resistance to high temperatures. By aligning the sequences of proteins from common ancestors (homologues) and different species, we are able to identify the protein building blocks (amino-acids) that are important to the fitness of a protein structure.
GPUs play a fundamental role in this as, thanks to the infrastructure in place, we are able to collect the large amount of data required to obtain statistical significance in the trends we observe. This is crucially important as having a high degree of confidence in our designs ensures that the experimental validation of the designed targets will be kept to a minimum of protein sequences. These sequences will therefore have a high likelihood to fit the purpose of the design, enormously reducing experimental costs by leaving the heavy lifting to the power of computational prediction.

Using pulsed electric fields to increase food safety
One of the largest problems in the New Zealand’s food industry is to keep food safe from the effects of pathogens, in particular a strain of E. coli producing a protein called Shiga toxin. Little is known about inactivating the toxin by using non-conventional food processing methods and most of the attention is indeed devoted to prevent the infection of cattle or meat by conventional antibacterial means, which, in turn, means preventing E. coli’s growth. We are focusing on an alternative strategy to inactivate toxins in food, by triggering the loss of a toxin’s structure by applying pulsed electric fields (PEFs). Applying PEFs consist of exposing food samples to short but powerful electric fields. Nevertheless, nothing is known regarding the effects of PEFs on the stability of proteins. We are performing simulations in which the pentameric Shiga toxin produced by E. coli is exposed to pulsed electric fields featuring different electric field strengths, frequencies and/or exposure times. In our simulations we then monitor the disrupting effects of the applied field on the toxin’s structure, which mediates its toxicity by binding receptors on the surface of human cells (Figure 2). Thus, by disrupting the toxin’s structure, its ability to interact with human cells and trigger a toxic response would be hindered. Overall, we thus plan to provide the industry with guidelines on what is required to inactivate the Shiga toxin, without affecting the organoleptic properties of meat. Interestingly, once this protocol is optimised for the Shiga toxin we will expand our study to other toxins, with the overarching goal of bringing the highly predictive potential of molecular simulations to service industry. Overall, we thus plan to provide the industry with guidelines on what is required to inactivate the Shiga toxin, without affecting the organoleptic properties of meat. Interestingly, once this protocol is optimised for the Shiga toxin we will expand our study to other toxins, with the overarching goal of bringing the highly predictive potential of molecular simulations to service industry.
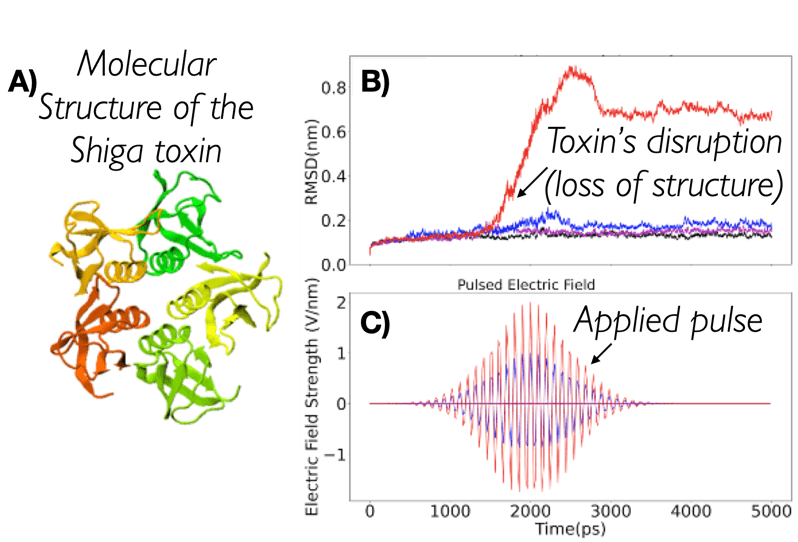
Rational design of molecular motors to obtain carbohydrates with desired physical properties
Great attention in biology is given to so-called molecular motors. Molecular motors are proteins that can accomplish multiple chemical reactions on other molecules (their substrates) in a highly efficient way. They can perform several reaction cycles without having to dissociate and reassociate from their substrate (for a new reaction cycle). This makes molecular motors very efficient, at the point that they are involved in a large number of fundamental biological processes. In the past, we have discovered a particular class of molecular called Pectin methyl esterase enzymes (PMEs), as they work on pectin. Pectin is a polysaccharide that is extremely important to maintaining the integrity of the plant cell wall and equally crucial to the food industry for its jellifying properties in the presence of calcium. Interestingly, the jellification properties of pectin are strictly related to PMEs, as they control the number of negatively charged sugars that promote jellification by binding positively charged calcium ions.
With an ad-hoc computational workflow we are therefore trying to answer the following question: is it possible to design new PMEs so to have a selected amount and distribution of negatively charged sugars and control the jellification properties of pectin for targeted uses in the food industry? Our intent for this project is to design PME enzymes that are able to provide pectin with defined physico-chemical properties, by modulating the charge distribution of pectic polysaccharides upon the modifications promoted by specifically engineered PMEs (Figure 3). Besides PMEs, their natural inhibitors (called PME inhibitors or PMEIs) are of paramount importance for the food industry as, by stopping the activity of PMEs in fruit, they can control fruit juice clarification, often hindered by overly charged pectin in juice. By increasing the thermal stability of PMEIs we are designing new inhibitors that can be applied in high-temperature food processing processes of fruit juice manufacturing.

Molecular simulations in the cloud: from highly parallelized to highly replicated runs
Molecular simulations aiming to sample the dynamics of molecular systems, either through Monte Carlo methods or integrating equations of motion over time, are heavily stochastic. The conformational space of even small molecules is enormous and computational sampling of the functional space (let alone the whole conformational space of a molecule) is extremely challenging. For the simulation of larger molecular systems, scalability of molecular dynamics codes allows us to split the computation, in other words the molecular system, across a large number of computing nodes that work in parallel. Molecular dynamics simulations are “infamous” for being heavily parallelised. However, besides the need to simulate ever-growing molecular systems (composed of several hundred thousand to millions of atoms), statistical robustness is also required to achieve confidence for the claims of a scientific study. To obtain this, it is a must to perform several molecular dynamics simulation runs (replicates), starting from slightly different initial conditions (i.e. the initial velocities assigned to the particles to simulate) and to monitor the evolution of the simulation, finally obtaining population-averaged via time-averaged results.
Replicates are virtually independent from each other, and for smaller molecular systems do not need a heavy parallelisation scheme or multiple nodes. Moreover, especially considering the power of GPU computing, longer timescales, which are directly linked to the investigation of molecular function, can be accessed. Overall, the need to simulate a large amount of replicated simulations, with small differences in the set of initial conditions, makes this problem highly suitable to be set up in a cloud-based infrastructure: with several replicates running on separate instances that do not communicate or work in parallel. With respect to these needs, Nectar instances are an invaluable resource for the understanding and design of molecular systems through molecular dynamics simulations.
What’s next?
There have been two recent hirings in the School of Chemical Sciences at the University of Auckland: one in the field of computational biophysics and another in physical chemistry with a particular focus on computational research. These have undoubtedly increased the need of computational resources deployed towards the study of fundamental chemistry and the design of new molecules optimised for industrial applications.
Cloud-based systems can play a fundamental role in this, provided that they would be able to overcome hurdles that often characterise the setup of new workflows. These hurdles can be mostly identified in two immediate needs: (1) the need to have automated workflows that allow the ready setup of molecular simulations and (2) the necessity to have a critical amount of GPU-accelerated instances that can promote competitive research.
While the former can be quite easily addressed by directing expertise towards the development of configuration scripts that would make simulations run smoothly on different instances, the latter is a matter of an economic nature, requiring strategic investments and tactical planning.
Initiatives directed towards the equipment of existing non-GPU accelerated instances with GPUs, the creation of hybrid infrastructures featuring both nVIDIA and AMD GPU instances and flexibility around the mainstream setups of data centres, are most likely key points to promote cost-effective but at the same time competitive research that can take enormous advantage from having a local GPU-enhanced computational infrastructure.
See more case study projects

Our Voices: using innovative techniques to collect, analyse and amplify the lived experiences of young people in Aotearoa
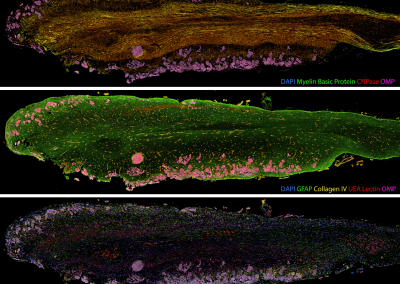
Painting the brain: multiplexed tissue labelling of human brain tissue to facilitate discoveries in neuroanatomy
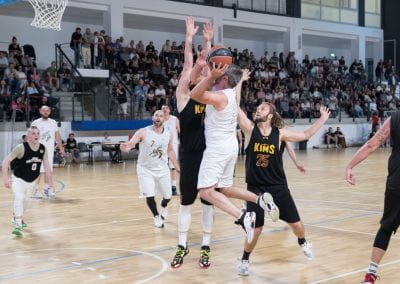
Detecting anomalous matches in professional sports: a novel approach using advanced anomaly detection techniques
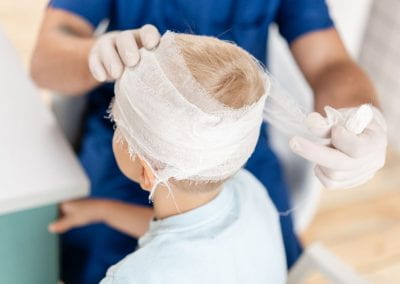
Benefits of linking routine medical records to the GUiNZ longitudinal birth cohort: Childhood injury predictors

Using a virtual machine-based machine learning algorithm to obtain comprehensive behavioural information in an in vivo Alzheimer’s disease model

Mapping livability: the “15-minute city” concept for car-dependent districts in Auckland, New Zealand

Travelling Heads – Measuring Reproducibility and Repeatability of Magnetic Resonance Imaging in Dementia
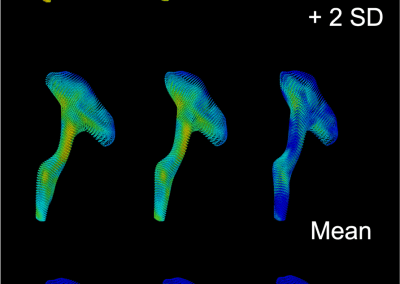
Novel Subject-Specific Method of Visualising Group Differences from Multiple DTI Metrics without Averaging

Re-assess urban spaces under COVID-19 impact: sensing Auckland social ‘hotspots’ with mobile location data

Aotearoa New Zealand’s changing coastline – Resilience to Nature’s Challenges (National Science Challenge)

Proteins under a computational microscope: designing in-silico strategies to understand and develop molecular functionalities in Life Sciences and Engineering
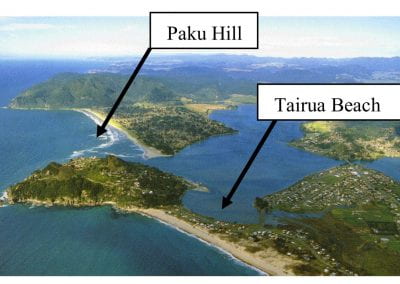
Coastal image classification and nalysis based on convolutional neural betworks and pattern recognition

Determinants of translation efficiency in the evolutionarily-divergent protist Trichomonas vaginalis
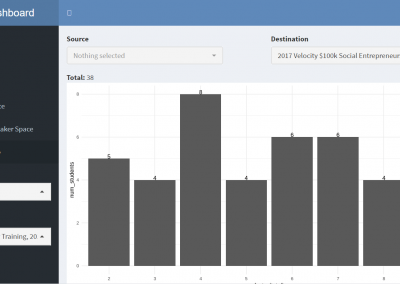
Measuring impact of entrepreneurship activities on students’ mindset, capabilities and entrepreneurial intentions
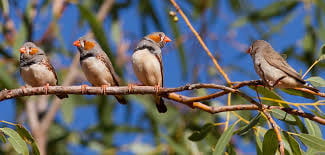
Using Zebra Finch data and deep learning classification to identify individual bird calls from audio recordings
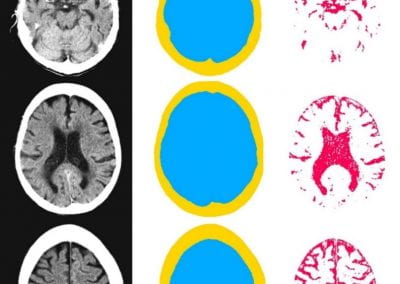
Automated measurement of intracranial cerebrospinal fluid volume and outcome after endovascular thrombectomy for ischemic stroke
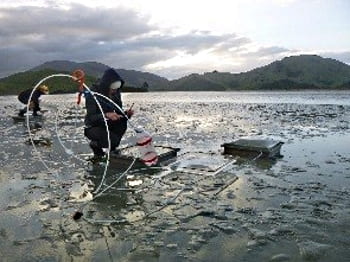
Using simple models to explore complex dynamics: A case study of macomona liliana (wedge-shell) and nutrient variations
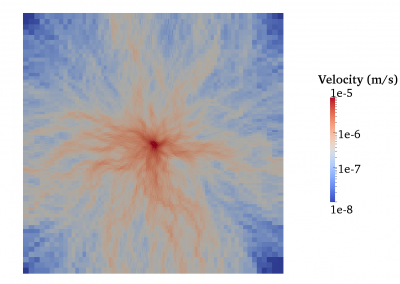
Fully coupled thermo-hydro-mechanical modelling of permeability enhancement by the finite element method
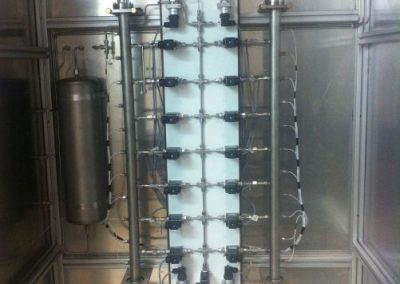
Modelling dual reflux pressure swing adsorption (DR-PSA) units for gas separation in natural gas processing
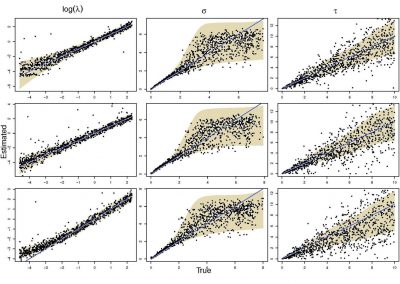
Molecular phylogenetics uses genetic data to reconstruct the evolutionary history of individuals, populations or species
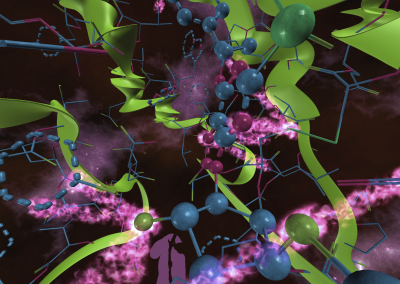
Wandering around the molecular landscape: embracing virtual reality as a research showcasing outreach and teaching tool
