
Towards the use of deep learning techniques for storm surge prediction
Ana Rueda, Universidad de Cantabria; Sina Masoud-Ansari, University of Auckland; Giovanni Coco, University of Auckland,; Laura Cagigal, University of Auckland / Universidad de Cantabria; Fernando Méndez, Universidad de Cantabria
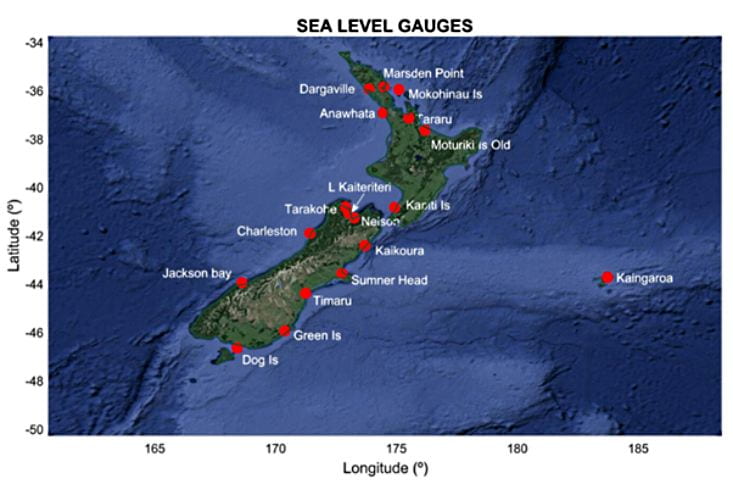
Figure 1. New Zealand sea level gauges, locations of New Zealand sea level gauges
Introduction
Accurate forecasts of extreme storm surge (SS) are important for port operation and coastal flooding early warning systems. To inform decision makers and managers two main approaches have been historically proposed: dynamical models and data-driven models. Dynamical models are more commonly used but the models are extremely computationally intensive and the methodology is usually applied to study specific locations or using very coarse resolution. In contrast, data-driven models look for statistical relations that link atmospheric variables and SS. Previous works applying statistical downscaling techniques have been found to properly reproduce SS at local and regional scales (Cid et al. (2016), French et al. (2017), Cagigal et al. (2019)). These previous works show high correlation and small errors between predicted and recorded SS. However, a slight underprediction on the higher quantiles of the surge distribution was also reported. This ongoing work is motivated by the recent development of deep learning techniques and by the lack of accuracy in the prediction of the most extreme events which, because of their association with the larger floods are of most concern.
Deep learning for storm surge prediction
The Center for eResearch collaborated with researchers in the School of Environment to develop a neural network to model the relationship between sea level pressure (SPL) fields and storm surge (SS) levels from a number of New Zealand tidal gauges (Fig. 1). The model consists of a convolutional component, which extracts spatial characteristics from the SLP fields and a recurrent component using on Long Short-Term Memory (LSTM) to model these characteristics over time (Hochreiter et al., 1997). The input to our model is the hourly SLP field in the area of influence and the predicted tide. The model was implemented using Python with Keras and Tensorflow with initial model training performed on the NeCTAR Research Cloud and subsequent model optimisation performed on New Zealand eScience Infrastructure (NeSI). Our preliminary results (Fig. 2) show the ability to learn SS behaviour. This project formed the basis for a recent MBIE Smart Ideas project that was awarded $1,000,000 to develop a production storm surge forecasting system in collaboration with MetService.

Figure 2. Preliminary results at Tararu showing the hourly true gauge level in meters (blue), model output during training (yellow) and model output during forecasting (green).
REFERENCES
- Cid, A., Camus, P., Castanedo, S., Méndez, F. J., & Medina, R. (2017). Global reconstructed daily surge levels from the 20th Century Reanalysis (1871–2010). Global and Planetary Change, 148, 9–21. https://doi.org/10.1016/j.gloplacha.2016.11.006
- Cagigal, L., Rueda, A., Castanedo, S., Cid, A., Perez, J., Coco, G., Mendez, F. (2019). Historical and Future Storm Surge around New Zealand: From the 19th century to the end of the 21st century. International Journal of climatology. 1-14. 10.1002/joc.6283
- French, J., Mawdsley, R., Fujiyama, T., Achutan, K., (2017). Combining machine learning with computationally hydrodynamics for prediction of tidal surge inundation at estuarine ports. IUTAM Symposium on Storm Surge Modelling and Forecasting.10.1016/j.piutam.2017.09.005
- Hochreiter, Sepp & Schmidhuber, Jürgen. (1997). Long Short-term Memory. Neural computation. 9. 1735-80.10.1162/neco.1997.9.8.1735.
See more case study projects

Our Voices: using innovative techniques to collect, analyse and amplify the lived experiences of young people in Aotearoa
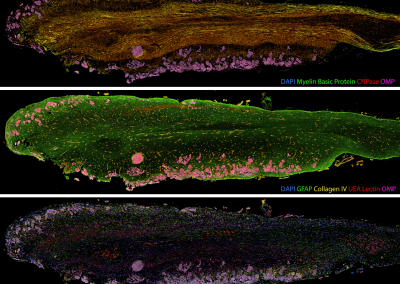
Painting the brain: multiplexed tissue labelling of human brain tissue to facilitate discoveries in neuroanatomy
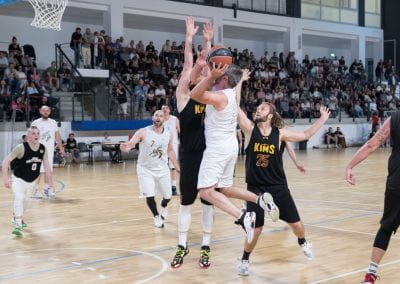
Detecting anomalous matches in professional sports: a novel approach using advanced anomaly detection techniques
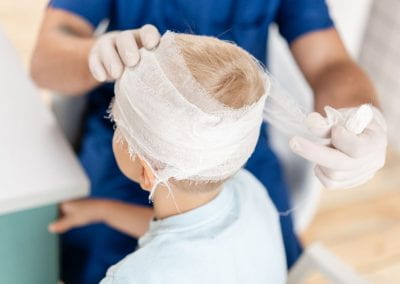
Benefits of linking routine medical records to the GUiNZ longitudinal birth cohort: Childhood injury predictors

Using a virtual machine-based machine learning algorithm to obtain comprehensive behavioural information in an in vivo Alzheimer’s disease model

Mapping livability: the “15-minute city” concept for car-dependent districts in Auckland, New Zealand

Travelling Heads – Measuring Reproducibility and Repeatability of Magnetic Resonance Imaging in Dementia
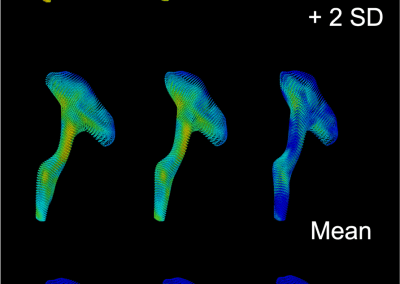
Novel Subject-Specific Method of Visualising Group Differences from Multiple DTI Metrics without Averaging

Re-assess urban spaces under COVID-19 impact: sensing Auckland social ‘hotspots’ with mobile location data

Aotearoa New Zealand’s changing coastline – Resilience to Nature’s Challenges (National Science Challenge)

Proteins under a computational microscope: designing in-silico strategies to understand and develop molecular functionalities in Life Sciences and Engineering
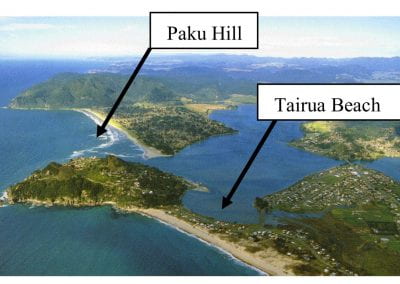
Coastal image classification and nalysis based on convolutional neural betworks and pattern recognition

Determinants of translation efficiency in the evolutionarily-divergent protist Trichomonas vaginalis
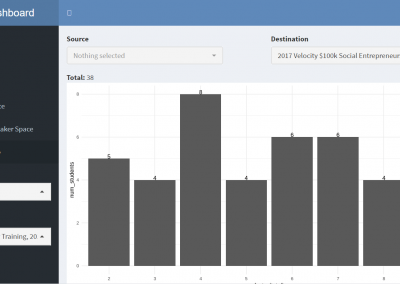
Measuring impact of entrepreneurship activities on students’ mindset, capabilities and entrepreneurial intentions
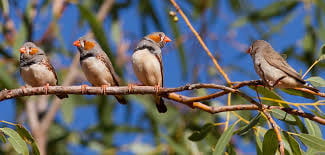
Using Zebra Finch data and deep learning classification to identify individual bird calls from audio recordings
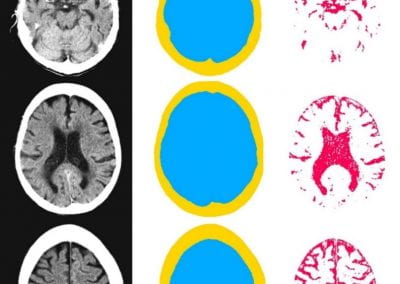
Automated measurement of intracranial cerebrospinal fluid volume and outcome after endovascular thrombectomy for ischemic stroke
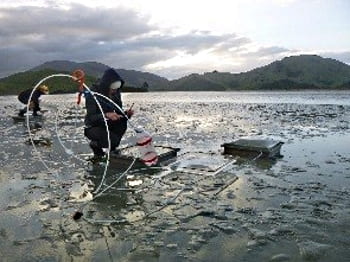
Using simple models to explore complex dynamics: A case study of macomona liliana (wedge-shell) and nutrient variations
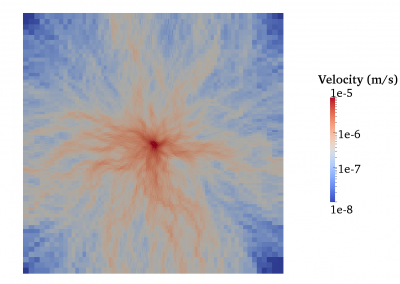
Fully coupled thermo-hydro-mechanical modelling of permeability enhancement by the finite element method
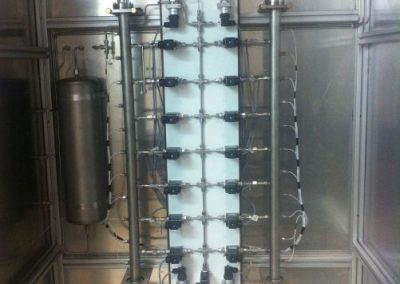
Modelling dual reflux pressure swing adsorption (DR-PSA) units for gas separation in natural gas processing
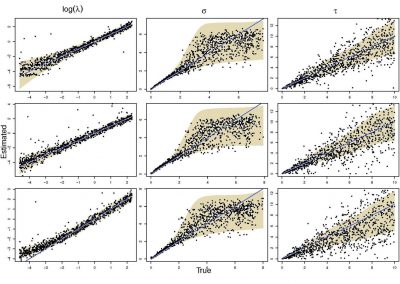
Molecular phylogenetics uses genetic data to reconstruct the evolutionary history of individuals, populations or species
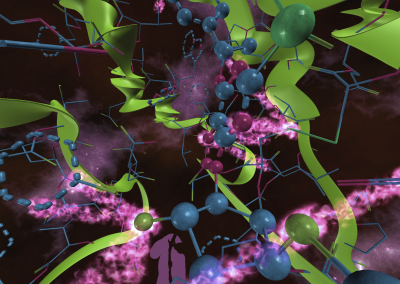
Wandering around the molecular landscape: embracing virtual reality as a research showcasing outreach and teaching tool
