
Understanding tumour evolution through augmented reality
Ben Lawrence, Tamsin Robb, Kate Parker, Braden Woodhouse, Faculty of Medical and Health Sciences, Mike Davis, Uwe Rieger, Jack Guo, School of Architecture & Planning, Jane Reeve, Auckland District Health Board
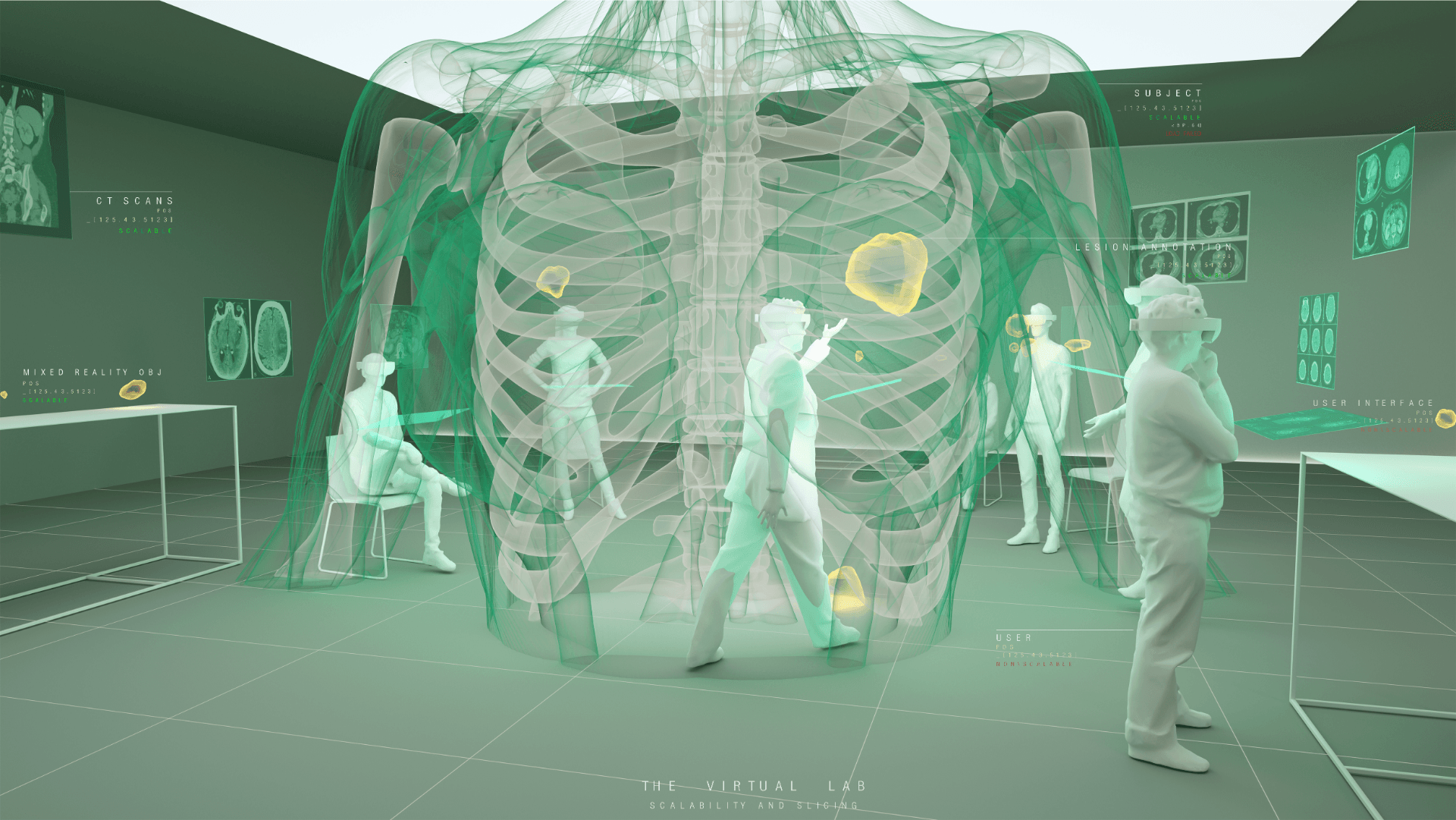
Introduction
The project presented a rare opportunity to study tumour evolution and heterogeneity in a single patient. The patient had a primary lung neuroendocrine tumour and 88 metastases, and requested and consented to rapid autopsy following a comprehensive ethical consultation with the patient and their extended family. We embarked on a multi-layered genomic investigation, utilising clinical notes and imaging in order to build a personalised evolutionary model of the disease progression. Initially 30 tumour samples, representing a broad spatial distribution of the patient’s total tumour burden, were analysed via DNA whole exome sequencing (WES), transcriptome mRNA sequencing and RNA microarray analysis, providing a bank of genomic information to guide evolutionary investigations. We have since built statistical phylogenetic models from differences observed across the genomic data to produce a broad evolutionary picture encompassing single nucleotide variants (SNVs), indels, copy number variants (CNVs), and RNA expression.
Augmented Reality (AR)
Understanding the complexities of genomic data coming from a multitude of spatially distinct sites, that further change across time, is a complex task. A novel approach was required to link spatial orientation to mutational patterns. A three-dimensional approach was preferred because of the regional complexities of sampling multiple sites within a single tumour, as well as from multiple tumour sites in a single patient. By using AR technology, the team has developed a 3D model of the patient’s tumours, the sampling sites, and representations of corresponding genomic data, generating a tool to better understand tumour evolution in a single patient.
Project collaboration
The project is a collaboration between the Centre for eResearch (CeR), School of Architecture & Planning (Open Media Lab) and the NETwork! project team from the Faculty of Medical and Health Sciences (FMHS). The team comprises of multidisciplinary expertise in different areas including the fields of clinical cancer care, cell biology, genomics (DNA / RNA data), histology (microscope slides), radiology (scans) and architecture and visualisation specialists.
The benefits of the model include pushing for conceptual change. This pilot will allow us to visualise tumours on a scale previously unachievable. It will enhance the understanding of tumour progression for a wider audience including patients and their families, as well as scientists, the research community and clinicians, and many more. We hope to break open the way scientists, clinicians and patients think about cancer, as well as enabling new conceptual connections.
Annotating sampling sites: Once the tumour meshes were constructed, Bianca worked with Tamsin Robb to translate information from the tumour collection process into accurate 3D sampling sites for visualisation in the HoloLens. At this stage, only genomic data collected from 30 sampling sites (of over 300 sites sampled) were included. We navigated to the point(s) in the tumours where incisions were made in order to best approximate sampling sites. Sampling sites were named and saved alongside the relative tumour mesh to be integrated later.
Assembling a skeletal framework: Fittingly, the patient’s own skeleton, produced from the most recent CT scans, was used as a frame of reference to place tumours and sampling sites in 3D space. Semi-automatic segmentation techniques were used to separate the bony components from soft organ tissue, completed by Bianca Haux. Manual editing was required to remove segmentation artefacts such as superimposed structures caused by the injection of contrast.
Proof of concept (6 months from March to October 2018)
Over this period, the team met weekly or fortnightly to work towards presenting these tumours in AR. The final product features a representation of the patient’s skeleton as the framework for all tumours (extracted from CT data). The model includes all tumours overlaid for multiple time-points following diagnosis, sampling sites for which we have genomic data indicated in space, and genomic groupings indicated with dynamic colouring options.
The process
In-HoloLens CT-annotation system: Nick Young from the CeR produced pseudo coloured 3D volumetric renders of CT scans that can be opened in the HoloLens, and a system for adding sphere annotations to the scans to allow an experienced radiologist or clinician to interpret CT scans in 3D and indicate tumour locations with 3D markers. We then proceeded to construct tumour meshes manually as following:
Constructing tumour meshes: In collaboration with radiologist Dr Jane Reeve, the team identified tumours on CT scans, and outlines were traced manually on the computer. These traces were used by Bianca Haux from the CeR to construct 3D models of each identified tumour from the scans. The term tumour “mesh” is used to describe a 3D model of the external shape of the tumour as taken from the clinical data.
In order to ensure users interacting with the model were left with the impression of a complete augmented reality patient rather than part-patient, Jack Guo attached default female skeletal limbs to the patient’s skeleton.
CT imaging: Skeletal, tumour and sampling site elements were combined in Unity, along with menus and controls needed to navigate the space, in order to produce an interactive 3D representation of the patient. Together with colour coding based on genomic data this allows users to study the possible progression of the patient’s disease over time and evolutionary space.
Developing “multiplayer”: One of the key reasons why AR technology was chosen for this model was the possibility to interact with the model in 3D space and, most importantly, with other users. Multiplayer was developed to enable this collaborative approach and encourage conversation and exchange of knowledge while looking at the 3 dimensional data. In a group setting this allows for users to point out, show and connect spatially relevant information with a tested group size of up to 6 people. The ability for users with different backgrounds and expertise to discuss and interact with the model in the same space and time allows for truly collaborative and novel thinking.
Other areas of concerns:
- Ethics: This work was conducted in close consultation with the patient’s family. We need to be vigilant of the high risk of patient identification. The team will continue to take all reasonable precautions to protect the patient’s privacy. The patient’s family are regularly updated on progress and have had a chance to see an early version of the AR model.
- System design: The team will look into a design that can support more than one brand of AR headset, so that it will be able to move with new technology when they become more widely available.
- Other ongoing questions include consideration of data flow, the usability test, the generation of IP, and building a one-off model or constructing a platform.
Next steps
After completion of Proof of Concept phase (over 6 months), we will then make future decisions based on outcomes of this pilot. We expect a considerable amount of circular thinking and regrouping. We are hoping to secure additional funding for the expansion of the project into further stages and determine a specific time point to make this decision.
See more case study projects

Our Voices: using innovative techniques to collect, analyse and amplify the lived experiences of young people in Aotearoa
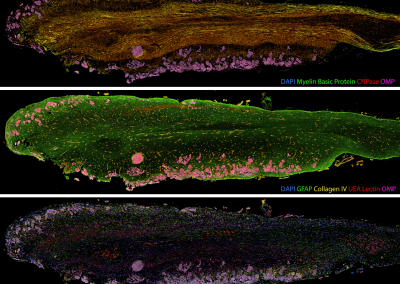
Painting the brain: multiplexed tissue labelling of human brain tissue to facilitate discoveries in neuroanatomy
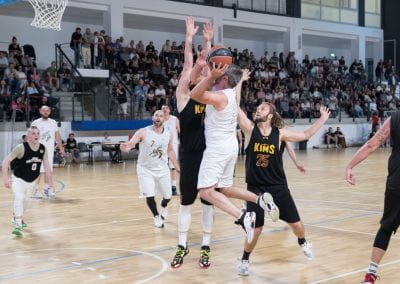
Detecting anomalous matches in professional sports: a novel approach using advanced anomaly detection techniques
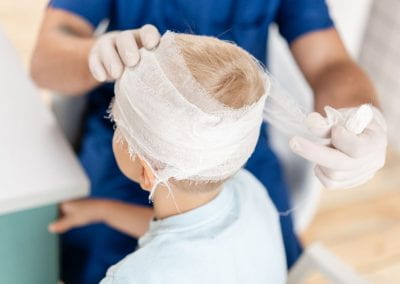
Benefits of linking routine medical records to the GUiNZ longitudinal birth cohort: Childhood injury predictors

Using a virtual machine-based machine learning algorithm to obtain comprehensive behavioural information in an in vivo Alzheimer’s disease model

Mapping livability: the “15-minute city” concept for car-dependent districts in Auckland, New Zealand

Travelling Heads – Measuring Reproducibility and Repeatability of Magnetic Resonance Imaging in Dementia
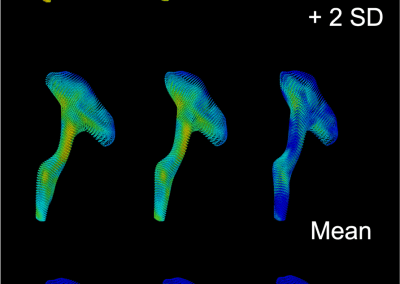
Novel Subject-Specific Method of Visualising Group Differences from Multiple DTI Metrics without Averaging

Re-assess urban spaces under COVID-19 impact: sensing Auckland social ‘hotspots’ with mobile location data

Aotearoa New Zealand’s changing coastline – Resilience to Nature’s Challenges (National Science Challenge)

Proteins under a computational microscope: designing in-silico strategies to understand and develop molecular functionalities in Life Sciences and Engineering
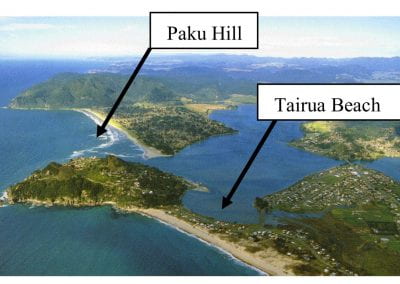
Coastal image classification and nalysis based on convolutional neural betworks and pattern recognition

Determinants of translation efficiency in the evolutionarily-divergent protist Trichomonas vaginalis
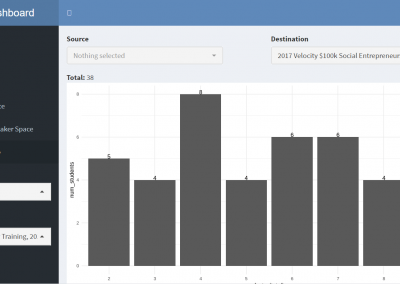
Measuring impact of entrepreneurship activities on students’ mindset, capabilities and entrepreneurial intentions
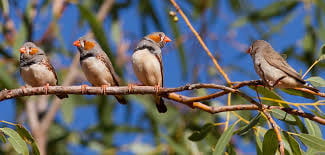
Using Zebra Finch data and deep learning classification to identify individual bird calls from audio recordings
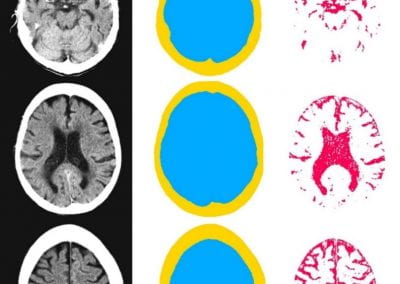
Automated measurement of intracranial cerebrospinal fluid volume and outcome after endovascular thrombectomy for ischemic stroke
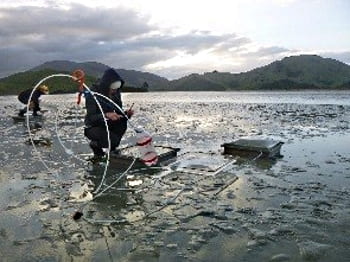
Using simple models to explore complex dynamics: A case study of macomona liliana (wedge-shell) and nutrient variations
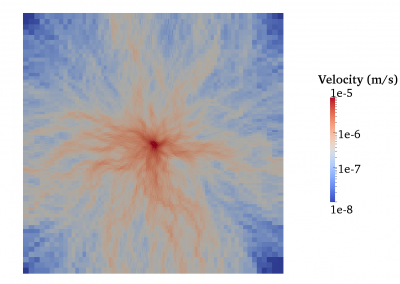
Fully coupled thermo-hydro-mechanical modelling of permeability enhancement by the finite element method
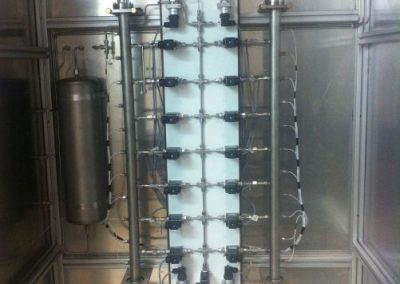
Modelling dual reflux pressure swing adsorption (DR-PSA) units for gas separation in natural gas processing
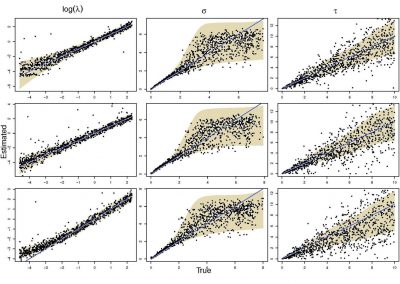
Molecular phylogenetics uses genetic data to reconstruct the evolutionary history of individuals, populations or species
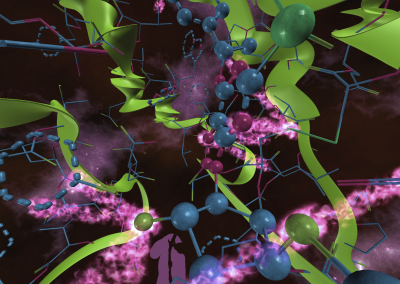
Wandering around the molecular landscape: embracing virtual reality as a research showcasing outreach and teaching tool
