
Taking a ‘Big Data’ approach to find new clinical-omic associations in cancer
Nicholas Knowlton, Peter Tsai and Professor Cris Print, Molecular Medicine and Pathology
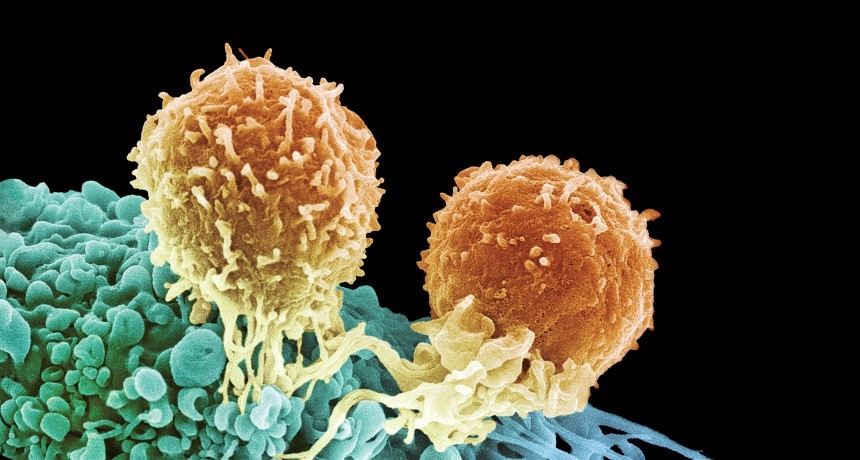
Cancer cells. Source: https://www.technicalforweb.com/wp-content/uploads/2017/04

Clinical-omics
Cancer is the number two cause of mortality in the OECD behind heart disease. Up until the late 1990’s, there was a concerted effort by drug companies to develop ‘blockbuster therapies’ for the treatment of cancer, i.e. cancer therapies developed with a one-size-fits-all approach. This philosophy was upended by the improvements in computer hardware and software that now allow scientists to link multiple types of genomic data about a given cancer together to identify patient-specific therapies.
Finding genomic link
In this study, we are using new statistical methods to find genomic links between a patient’s genomic immune signature and clinical information across multiple types of cancer. This will help identify the types of cancer patient who can benefit from the new ‘Immune Checkpoint Inhibitor’ drugs, which activate the patient’s own immune system to kill cancer cells.
Currently, these drugs are only effective in a subset of patients – we are working towards identifying why this is and how to predict which patients will respond. We are accessing a 2.5 petabyte international genomic data archive of over 11 000 patients in an attempt to identify clinically useful but previously undiscovered information about cancers.
To identify a potential antigens (altered proteins on the surface of cancer cells encoded by mutant genes), we download a patient’s raw tumour gene sequence along with a normal tissue gene sequence and extract out the portion of the genome responsible for creating an immune response. Next, we use computer algorithms to compute the likely affinity between novel antigens presented by each patient’s individual cancer and the individual patient’s genomic immune signature. The initial results are promising, by simply counting the number of novel antigens predicted in 491 melanoma patients a survival difference is identified, where more mutations in individual tumours leads to a greater immune response by the patient’s body against the tumour cells and longer patient survival (Figure 1).
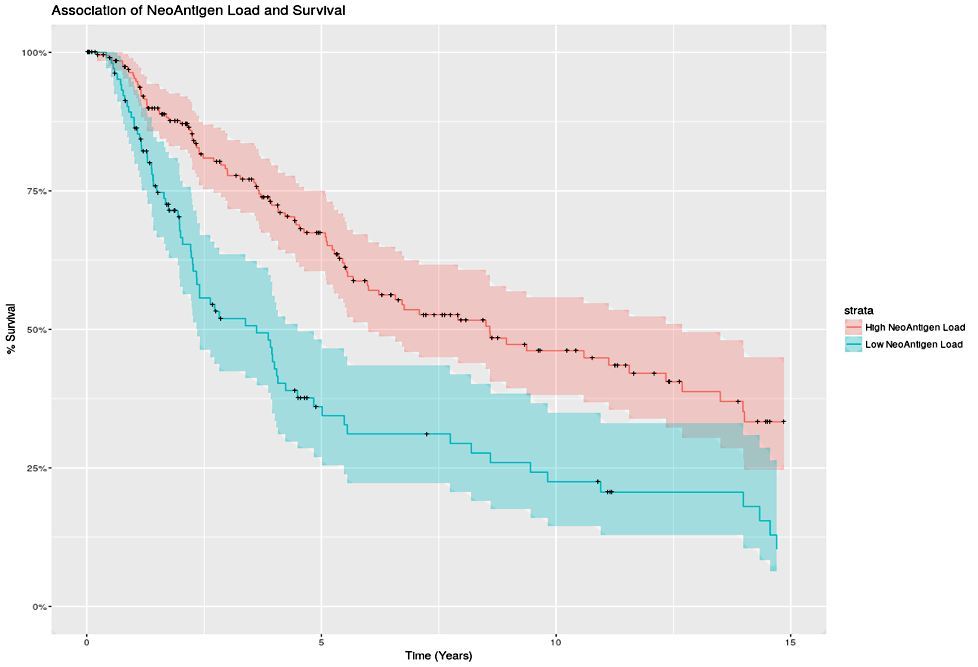
Figure 1. Association of NeoAntigen Load and Survival.
Data transfer, analysis and storage
We worked with the Centre for eResearch to develop and host the download of data from GDC secure servers, run analysis and access long term storage using a research virtual machine (RVM) running and research storage. This provided the essential compute, memory and network needs to process hundreds of terabytes of information down into digestible chunks. One advantage of the RVM 24/7 access to a dedicated 1 GB network link to download the necessary data. For example, processing a single tumour type it takes approximately 192 hours when including time to download and extract the relevant data. This is followed by an additional 60 hours to predict possible interactions of novel antigens in the tumours.
The future
The results of the initial analysis are promising and we will continue to process additional cancer types that will enable us to perform a pan-cancer analysis of computationally predicted novel antigens. This work is supported by the Maurice Wilkins Centre of Research Excellence.
See more case study projects

Our Voices: using innovative techniques to collect, analyse and amplify the lived experiences of young people in Aotearoa
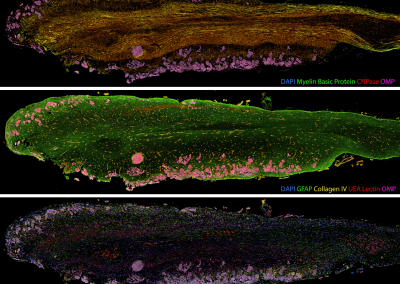
Painting the brain: multiplexed tissue labelling of human brain tissue to facilitate discoveries in neuroanatomy
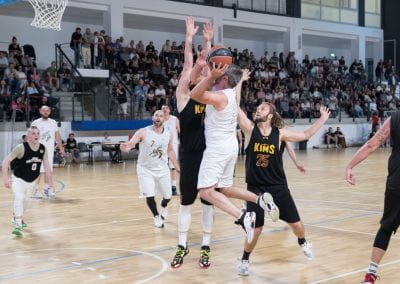
Detecting anomalous matches in professional sports: a novel approach using advanced anomaly detection techniques
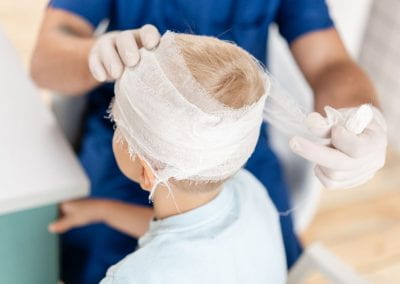
Benefits of linking routine medical records to the GUiNZ longitudinal birth cohort: Childhood injury predictors

Using a virtual machine-based machine learning algorithm to obtain comprehensive behavioural information in an in vivo Alzheimer’s disease model

Mapping livability: the “15-minute city” concept for car-dependent districts in Auckland, New Zealand

Travelling Heads – Measuring Reproducibility and Repeatability of Magnetic Resonance Imaging in Dementia
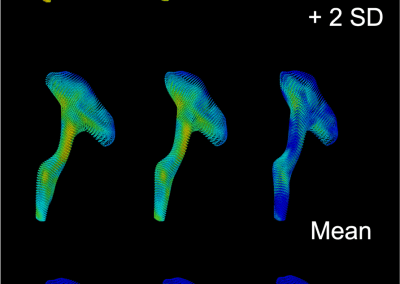
Novel Subject-Specific Method of Visualising Group Differences from Multiple DTI Metrics without Averaging

Re-assess urban spaces under COVID-19 impact: sensing Auckland social ‘hotspots’ with mobile location data

Aotearoa New Zealand’s changing coastline – Resilience to Nature’s Challenges (National Science Challenge)

Proteins under a computational microscope: designing in-silico strategies to understand and develop molecular functionalities in Life Sciences and Engineering
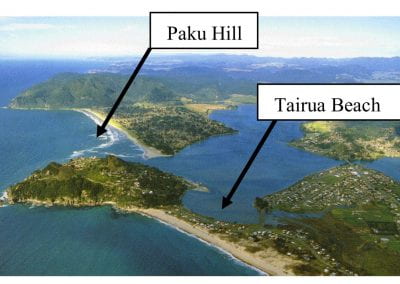
Coastal image classification and nalysis based on convolutional neural betworks and pattern recognition

Determinants of translation efficiency in the evolutionarily-divergent protist Trichomonas vaginalis
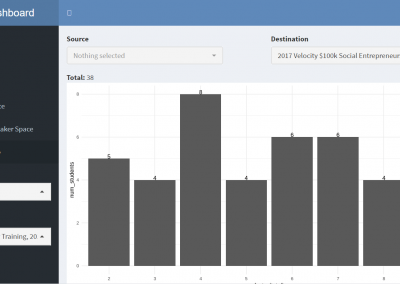
Measuring impact of entrepreneurship activities on students’ mindset, capabilities and entrepreneurial intentions
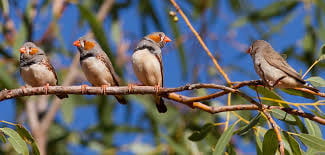
Using Zebra Finch data and deep learning classification to identify individual bird calls from audio recordings
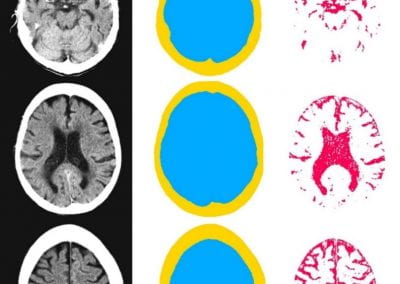
Automated measurement of intracranial cerebrospinal fluid volume and outcome after endovascular thrombectomy for ischemic stroke
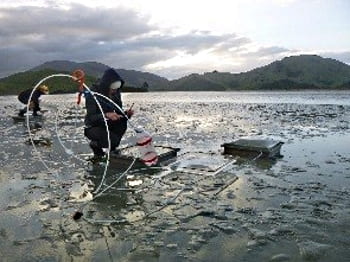
Using simple models to explore complex dynamics: A case study of macomona liliana (wedge-shell) and nutrient variations
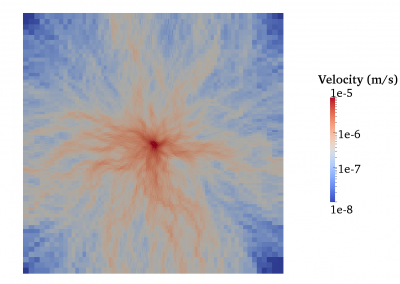
Fully coupled thermo-hydro-mechanical modelling of permeability enhancement by the finite element method
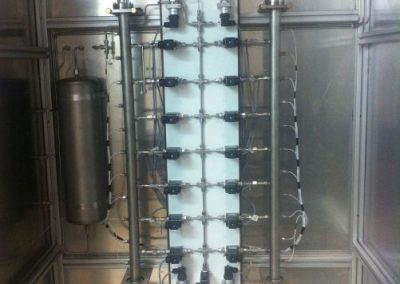
Modelling dual reflux pressure swing adsorption (DR-PSA) units for gas separation in natural gas processing
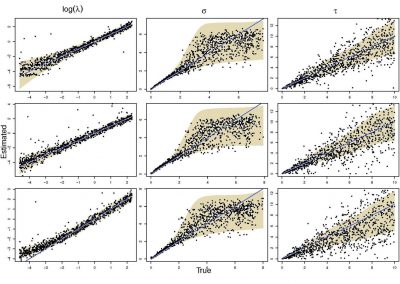
Molecular phylogenetics uses genetic data to reconstruct the evolutionary history of individuals, populations or species
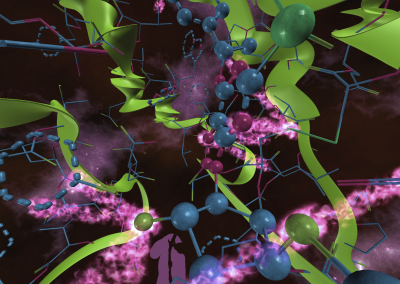
Wandering around the molecular landscape: embracing virtual reality as a research showcasing outreach and teaching tool
